Dijkstraโs Algorithm
2020-1ํ๊ธฐ, ๋ํ์์ โ์๊ณ ๋ฆฌ์ฆโ ์์ ์ ๋ฃ๊ณ ๊ณต๋ถํ ๋ฐ๋ฅผ ์ ๋ฆฌํ ๊ธ์ ๋๋ค. ์ง์ ์ ์ธ์ ๋ ํ์์ ๋๋ค :)
<๋ค์ต์คํธ๋ฅด๋ผ ์๊ณ ๋ฆฌ์ฆ; Dijkstraโs Algorithm>์ ๊ทธ๋ํ์์ ์ต๋จ ๊ฒฝ๋ก๋ฅผ ์ฐพ๋ ๋ํ์ ์ธ ์๊ณ ๋ฆฌ์ฆ์ด๋ค. ๊ทธ๋ํ์ ๋ชจ๋ weight๊ฐ ๋์ผํ ๊ฐ์ ๊ฐ์ง๋ค๋ฉด, BFS๋ฅผ ํตํด์ ์ต๋จ ๊ฒฝ๋ก๋ฅผ ๊ตฌํ ์ ์๋ค. ๋๋ ๊ฐ edge์ weight ๊ฐ์ด ๋ค๋ฅด๋๋ผ๋, ์๋์ ๊ฐ์ด dummy node๋ฅผ ์์ฑํ๋ค๋ฉด, BFS๋ก๋ ์ถฉ๋ถํ ์ต๋จ ๊ฒฝ๋ก๋ฅผ ์ป์ ์ ์๋ค.
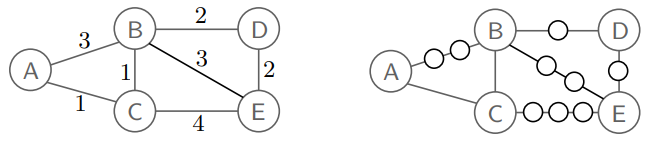
ํ์ง๋ง, ๋ง์ฝ $\max w_i$์ ๊ฐ์ด 10,000์ผ ์ ๋๋ก ์ ๋ง ํฐ ๊ฐ์ด๋ผ๋ฉด, ์ฐ๋ฆฌ๋ dummy node๋ฅผ ์์ฑํ๋ ๋ฐ์ ๋๋ฌด ๋ง์ overhead๋ฅผ ์ฐ๊ฒ ๋๋ค.
Dijsktraโs Algorithm
BFS์ ์ด๋ฐ ๋ฌธ์ ๋ฅผ ํด๊ฒฐํ๊ธฐ ์ํด <alarm clock algorithm>์ ๋์ ํ ์ ์๋ค.
1. Set $\texttt{alaram}(s)=0$, and for all other nodes $v$, set $\texttt{alarm}(v)=\infty$.
2. Repeat until thereโre no more alarms:
2.1. Say the next alarm rings at time $T$, for node $u$. Then, the distance from $s$ to $u$ is $T$.
2.2. For each neighbor $v$ of $u$, $\texttt{alarm}(v) = \min \left\{ \texttt{alarm}(v), T + \ell (u, v) \right\}$
์์์ ์ ์๋ <alarm clock algorithm>์ <์ฐ์ ์์ ํ; priority queue>๋ฅผ ํตํด ์ฝ๊ฒ ๊ตฌํํ ์ ์๋ค!
Algorithm: dijkstra($G$, $\ell$, $s$)
โป ์ฃผ์: ๊ทธ๋ํ $G$์ ๋ชจ๋ edge๋ positive edge์ฌ์ผ ํ๋ค.
// initialization
for all $u \in V$ do
โโ $\texttt{dist}(u) = \infty$
โโ $\texttt{prev}(u) = \texttt{nil}$
end for
// construct heap
$\texttt{dist}(s) = 0$
$H = \texttt{makequeue}(V)$
// explore frontier!
while $H$ is not empty do
โโ $u = \texttt{deletemin}(H)$
โโ for all edges $(u, v) \in E$ do
โโโโ if $\texttt{dist}(v) > \texttt{dist}(u) + \ell(u, v)$ then
โโโโโโ $\texttt{dist}(v) = \texttt{dist}(u) + \ell(u, v)$
โโโโโโ $\texttt{prev}(v) = u$
โโโโโโ $\texttt{decreasekey}(H, v)$
โโโโend if
โโend for
end while
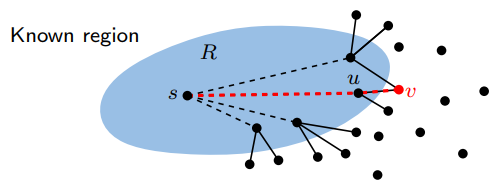
๋ค์ต์คํธ๋ผ ์๊ณ ๋ฆฌ์ฆ์ Explored ์์ญ์์ Frontier ์์ญ์ ํ์ํ๊ณ ํ์ฅํ๋ ๋ฐฉ์์ผ๋ก ๋์ํ๋ค.
๊ฐ๋จํ๊ฒ ์๊ฐํ๋ฉด, shortest path๋ก ์๋ดํ๋ <shortest path tree>๋ฅผ ๊ตฌ์ถํ ๋, ์ด๋ฏธ ์์ฑ๋ shortest path tree์ ์ธ์ ํ ๋ ธ๋๋ค์ธ Frontier๋ฅผ ์ดํด๋ณด๋ฉฐ, SPT๋ฅผ ํ์ฅํ๋ค๋ ๋ง์ด๋ค. ์ด๋, SPT growing rule์ ํ์ฌ์ heap์์ ๊ฐ์ฅ ์์ cost๋ฅผ ๊ฐ์ง๋ ๋ ธ๋๋ฅผ ๊ธฐ์ค์ผ๋ก ํ์ฅ์ ํ๋ค๋ ๊ฒ์ด๋ค!
์์ ๊ฐ์ ๋ฐฉ์์ผ๋ก ์ ๊ทผํ์ฌ ์์์ ์๊ฐํ ๋ฐฉ์์ ๋์น์ธ ์๊ณ ๋ฆฌ์ฆ์ ์ ์ํ ์๋ ์๋ค.
Algorithm: dijkstra($G$, $\ell$, $s$)
// initialization
for all $u \in V$ do
โโ $\texttt{dist}(u) = \infty$
โโ $\texttt{prev}(u) = \texttt{nil}$
end for
// construct heap
$\texttt{dist}(s) = 0$
$R = \left\{ \right\}$ (the โknown regionโ)
// explore frontier!
while $R \ne V$ do
โโ Pick the node $v \notin R$ with smallest $\texttt{dist}$ value
โโ Add $v$ to $R$
โโ for all edges $(v, z) \in E$
โโโโ if $\texttt{dist}(z) > \texttt{dist}(v) + \ell(v, z)$ then
โโโโโโ $\texttt{dist}(z) = \texttt{dist}(v) + \ell(v, z)$
โโโโend if
โโend for
end while
Complexity
๋น์ฐํ๊ฒ๋ ๋ค์ต์คํธ๋ผ ์๊ณ ๋ฆฌ์ฆ์ BFS๋ณด๋ค ๋ ๋๋ฆฌ๋ค. ์๋ํ๋ฉด, Priority Queue์ ์ฐ์ฐ์ด ๋ค์ด๊ฐ๊ธฐ ๋๋ฌธ์ด๋ค. (PQ์ ์ฐ์ฐ์ ๊ธฐ๋ณธ $\log_2 n$๋ ๋จน๋๋ค.)
๋ค์ต์คํธ๋ผ๋ ๊ธฐ๋ณธ์ ์ผ๋ก ๋ชจ๋ ๋ ธ๋๋ฅผ ์ดํด๋ณด๊ธฐ ๋๋ฌธ์, ๋ ธ๋ ๊ฐฏ์์ธ $\left| V \right|$๋ฒ ๋งํผ PQ์ $\texttt{insertion}$ ํ๊ฒ ๋๋ค.
๋, PQ๋ฅผ ๋น์ฐ๊ธฐ ์ํด PQ์ ๋ฃ์ ๋ ธ๋๋ฅผ $\texttt{deletemin}$์ผ๋ก ํ๋ฒ์ฉ ๋ชจ๋ ๊บผ๋ด์ค์ผ ํ๊ธฐ ๋๋ฌธ์ $\left| V \right|$๋ฒ ๋งํผ PQ์ $\texttt{deletemin}$ ํ๊ฒ ๋๋ค.
๊ทธ๋ฆฌ๊ณ ๊ฐ fronter point์์ ๋ ธ๋์ ์ฐ๊ฒฐ๋ ๋ชจ๋ edge๋ฅผ ์ดํด๋ณด๊ธฐ ๋๋ฌธ์ $\left| E \right|$๋ฒ ๋งํผ $\texttt{decreasekey}$ ์ฐ์ฐ์ ์ํํ๋ค.
๋ง์ฝ Binary Heap๋ก ๊ตฌํ๋ PQ๋ฅผ ์ฌ์ฉํ๋ค๋ฉด,
- $\texttt{deletemin}$์ $O(\log \left| V \right|)$ ๋งํผ์ ๋น์ฉ์ด ๋ค๊ณ ,
- $\texttt{insert}$์ $\texttt{decreasekey}$๋ $O(\log \left| V \right|)$ ๋งํผ์ ๋น์ฉ์ด ๋ ๋ค.
๋ฐ๋ผ์, ์ ์ฒด ๋น์ฉ์
\[O(V \log V) + O((V + E) \log V) = O((V+E) \log V)\]PQ ๋๋ Heap์ Binary Heap์ด ์๋ ๋ค๋ฅธ ๋ฐฉ์๋ค, ์๋ฅผ ๋ค๋ฉด, <$d$-ary heap>, <Fibonacci heap> ๋ฑ์ผ๋ก ๊ตฌํํด ์๊ฐ์ ๋ ์ค์ผ ์๋ ์๋ค๊ณ ํ๋ค.
* ์์ธํ ๋ด์ฉ์ ์ํคํผ๋์์ ํด๋น ํญ๋ชฉ์ ์ฐธ๊ณ ๐ link
์ถ๊ฐ ํด์ค
โป Note: Dijkstraโs Algorithm์ <UCS; Universal Cost Search>๋ผ๊ณ ๋ ๋ถ๋ฅธ๋ค.
Q. UCS๋ ์ ๋ง minimum cost path๋ฅผ ๋ณด์ฅํ๋๊ฐ?
โ ์ฆ, PQ์์ $\texttt{pop}$๋๋ ๋ ์์ ๊ทธ๋์ $\texttt{PastCost}(s)$ minimum cost์์ ๋ณด์ฅํ๋ค.
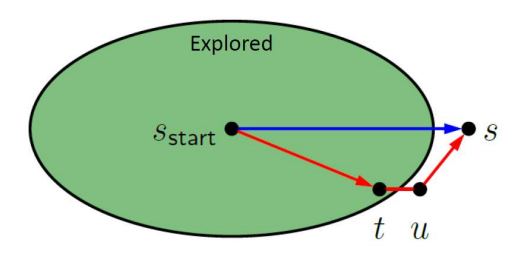
A. (๊ท๋ฅ๋ฒ) $s$๊ฐ PQ์์ $\texttt{pop}$๋ ๋, ๊ทธ๋์ $\texttt{PastCost}(s)$๊ฐ minimum cost๊ฐ ์๋๋ผ๊ณ ๊ฐ์ ํ์. ์ด๊ฒ์ $s$ ์ดํ์ $\texttt{pop}$๋๋ $u$๋ผ๋ ์ด๋ค ๋ ธ๋๊ฐ ์๊ณ , $u \rightarrow s$๋ก ๊ฐ๋ path๊ฐ minimum cost๋ฅผ ๊ฐ์ง์ ์๋ฏธํ๋ค.
ํ์ง๋ง, PQ๋ $\texttt{PastCost}(\cdot)$์ด ์์ ์์๋๋ก ์ ๋ ฌํ๊ธฐ ๋๋ฌธ์ ์ดํ์ $\texttt{pop}$๋๋ $u$์ $\texttt{PastCost}(u)$๋ $\texttt{PastCost}(s)$๋ณด๋ค ํด ๊ฒ์ด๋ค. ์ด๊ฒ์ $\texttt{PastCost}(u) + \ell(u, s) < \texttt{PastCost}(s)$๋ผ๋ $u$์ ์กด์ฌ์ ๋ชจ์๋๋ค. ๊ทธ๋์ $u$๋ฅผ ๊ฑฐ์ณ $s$๋ก ๊ฐ๋ path๋ ์ ๋ minimum cost path๊ฐ ๋ ์ ์๋ค.
๋ฐ๋ผ์ $s$๊ฐ $\texttt{pop}$๋ ๋์ $\texttt{PastCost}(s)$๋ณด๋ค ์์ minimum cost path๋ ์กด์ฌํ ์ ์์ผ๋ฏ๋ก $s$๊ฐ $\texttt{pop}$๋ ๋์ $\texttt{PastCost}(s)$๊ฐ minimum cost์ด๋ค.
Q. ์ UCS๋ negative cost๋ฅผ ์ง์ํ์ง ์๋๊ฐ?
A. ๋ง์ฝ negative cost๊ฐ ์กด์ฌํ๋ค๋ฉด, PQ๋ฅผ ์ด์ฉํด min-cost tree๋ฅผ ๋ง๋๋ ๊ณผ์ ์์ ์ด๋ฏธ ํ์์ ์๋ฃํ Explored ๋ ธ๋์ min-cost๊ฐ ๋ฐ๋ ์ ์๊ธฐ ๋๋ฌธ์ ์ด๋ฏธ ๊ตฌ์ถํ min-cost tree๋ฅผ ๋ฌด๋๋จ๋ฆฌ๊ฒ ๋๋ค. ์ด๊ฒ์ ์์ ๋ช ์ ์์ ์ธ๊ธํ โ์ดํ์ $\texttt{pop}$๋๋ $u$์ $\texttt{PastCost}(u)$๋ $\texttt{PastCost}(s)$๋ณด๋ค ํด ๊ฒ์ด๋ค.โ๋ผ๋ ๋ช ์ ๋ฅผ ์๋ฐํ๋ ๊ฒ์ด๊ณ , ๋์ด์ UCS์ correctness๋ฅผ ๋ณด์ฅํ ์ ์๋ค๋ ๋ง์ด ๋๋ค.
<๋ค์ต์คํธ๋ผ ์๊ณ ๋ฆฌ์ฆ>์ ๋จ์ ์ Negative Edge๋ฅผ ์ฒ๋ฆฌํ์ง ๋ชปํ๋ค๋ ๊ฒ์ด๋ค. ์ด์ด์ง๋ ํฌ์คํธ์์๋ Negative Edge๋ฅผ ์ฒ๋ฆฌํ๋ ์๊ณ ๋ฆฌ์ฆ์ธ <Bellman-Ford Algorithm>์ ๋ํด ์ดํด๋ณธ๋ค.