Chi-square Goodness-of-fit Test
โํ๋ฅ ๊ณผ ํต๊ณ(MATH230)โ ์์ ์์ ๋ฐฐ์ด ๊ฒ๊ณผ ๊ณต๋ถํ ๊ฒ์ ์ ๋ฆฌํ ํฌ์คํธ์ ๋๋ค. ์ ์ฒด ํฌ์คํธ๋ Probability and Statistics์์ ํ์ธํ์ค ์ ์์ต๋๋ค ๐ฒ
<Proportion Test>์ ๋ด์ฉ์ ๋จผ์ ์ดํด๋ณด๊ณ ์ค๋ ๊ฒ์ ์ถ์ฒํ๋ค. <Proportion Test>๋ฅผ ์ผ๋ฐํํ ๊ฒ์ด <Goodness-of-fit Test>์ด๊ธฐ ๋๋ฌธ์ด๋ค!
Introduction to Goodness-of-fit Test
<Goodness-of-fit Test; ์ ํฉ๋ ๊ฒ์ >์ population distribution์ด categorical variable์ ๊ฐ์ง๋ ๊ฒฝ์ฐ, ์๋ฅผ ๋ค์ด Head-Tail์ ๋์ ๋์ง๊ธฐ, ์ฃผ์ฌ์ ๋์ง๊ธฐ ๋ฑ์์ ์ฌ์ฉํ๋ ๊ฒ์ ๊ธฐ๋ฒ์ด๋ค. <Goodness-of-fit Test>๋ ์นดํ ๊ณ ๋ฆฌ ๋ณ์์ Sample Distribution (๋๋ Observed Distribution)์ด ๊ฐ์ ํ Expected Distribution๊ณผ ์ผ์นํ๋์ง๋ฅผ ๊ฒฐ์ ํ๋ค.
๋จผ์ ์๋์ ์์ ๋ฅผ ํ๋ฉด์, <Goodness-of-fit Test; ์ ํฉ๋ ๊ฒ์ >์ ๋ํด ์ดํด๋ณด์.
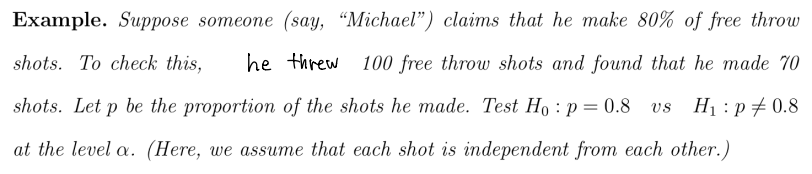
1. ๊ฒ์ ์ ๋ชฉํ
- $H_0: p=0.8$
- $H_1: p \ne 0.8$
- significance level $\alpha$
2. ์ํ๋ง ์ํฉ
- | made | missed | total |
---|---|---|---|
observed | 70 | 30 | 100 |
expected under $H_0$ |
80 | 20 | 100 |
3. Test Statistic
์ด์ ๊ฒ์ ์ ์ํํ๊ธฐ ์ํ <Test Statistic>์ ๊ฒฐ์ ํ์. sample proportion $\hat{p}$๋ฅผ ์ฌ์ฉํ๋ค.
$\hat{p}$์ CLT๋ฅผ ์ ์ฉํ๋ฉด, ์๋์ ๊ฐ๋ค.
\[\frac{\hat{p} - p}{\sqrt{p(1-p) / n}} \sim N(0, 1)\]์ด์ ์ <Proportion Test>์์ ์ด๊ฑธ ๊ทธ๋๋ก ์ฌ์ฉํ๋ค.
\[\text{reject} \; H_0, \quad \text{if} \quad \left| \frac{\hat{p} - p}{\sqrt{p(1-p) / n}} \right| > z_{\alpha/2}\]chi-square test์์ z-value์ ์ ๊ณฑ์ ์ทจํ๋ค.
\[\text{reject} \; H_0, \quad \text{if} \quad \left| \frac{\hat{p} - p}{\sqrt{p(1-p) / n}}\right|^2 > \left| z_{\alpha/2}\right|^2 = \chi^2_{\alpha}(1)\]<Goodness-of-fit Test>๋ฅผ ์๊ฐํ ๋๋ ๋งํ๋ฏ์ด <Goodness-of-fit Test>๋ ์นดํ ๊ณ ๋ฆฌ ๋ณ์์ ๋ํ ๊ฒ์ ์ด๋ค. ์์ ์์ ์นดํ ๊ณ ๋ฆฌ๊ฐ 2๊ฐ ๋ฟ์ธ ์ํฉ์์๋ง ์ฑ๋ฆฝํ๋ค. ๊ทธ๋์ ์์ ์์ ์ฝ๊ฐ ๋ณํํด <GOF Test>์ ์์ ์ ๋ํด๋ณด์.
์ผ๋จ์ 2๊ฐ ์นดํ ๊ณ ๋ฆฌ์์ ์์ํด๋ณด์.
\[\begin{aligned} \left| \frac{\hat{p} - p}{\sqrt{p(1-p) / n}}\right|^2 &= \frac{(\hat{p} - p)^2}{p(1-p)/n} \\ &= \frac{(x/n - p)^2}{p(1-p)/n} \\ &= \frac{(x/n - p)^2 \times n^2}{p(1-p)/n \times n^2} \\ &= \frac{(x - np)^2}{np(1-p)} \end{aligned}\]$\dfrac{1}{y(1-y)} = \dfrac{1}{y} + \dfrac{1}{1-y}$์์ ์ด์ฉํด ์์ ์๋์ ๊ฐ์ด ๋ถํดํ๋ค.
\[\begin{aligned} \frac{(x - np)^2}{np(1-p)} &= \frac{(x-np)^2}{np} + \frac{(x-np)^2}{n(1-p)} \end{aligned}\]์ด๋, $np$๋ ์ฒซ๋ฒ์งธ ์นดํ ๊ณ ๋ฆฌ์ ๋ํ expected value์ธ $e_1 = 80$์ด๊ณ , $n(1-p)$๋ ๋๋ฒ์งธ ์นดํ ๊ณ ๋ฆฌ์ ๋ํ $e_2 = 20$์ด๋ค. ๋ง์ฐฌ๊ฐ์ง๋ก, ๋ถ์์ $(x-np)^2$๋ โobserved value์ expected value์ ์ฐจ์ด ๊ฐโ์ด๋ค.
\[(x-np)^2 = (o_1 - e_1)^2\]๊ทธ๋ฐ๋ฐ $(x-np)^2$๋ฅผ ์๋์ ๊ฐ์ด ํํํ๋ฉด, ๋๋ฒ์งธ observed value์ expected value์ ์ฐจ์ด ๊ฐ์ผ๋ก ํํํ ์๋ ์๋ค!
\[(x-np)^2 = \left( (x-n) + (n-np) \right)^2 = (o_2 - e_2)^2\]์์ ์ข ํฉํ๋ฉด ์๋์ ๊ฐ๊ณ ,
\[\left| \frac{\hat{p} - p}{\sqrt{p(1-p) / n}}\right|^2 = \frac{(o_1 - e_1)^2}{e_1} + \frac{(o_2 - e_2)^2}{e_2}\]rejection criterion์ ๋ค์ ์ฐ๋ฉด,
\[\text{reject} \; H_0, \quad \text{if} \quad \sum_{i=1}^2 \frac{(o_i - e_i)^2}{e_i} > \chi^2_{\alpha}(1)\]2๊ฐ ์นดํ ๊ณ ๋ฆฌ ์์ ๋ฅผ $k$๊ฐ ์นดํ ๊ณ ๋ฆฌ๋ก ์ผ๋ฐํ ํ์ฌ ๊ธฐ์ ํด๋ณด์.
Definition. Test Statistic for Goodness-of-fit
<Goodness-of-fit>์ Test Statistic์
\[\chi^2 := \sum_{i=1}^k \frac{(o_i - e_i)^2}{e_i}\]where $o_i$ and $e_i$ are the observed and expected occurrences respectively.
๐ฅ NOTE: all expected occurrences must be at least 5. ๋ง์ฝ, 5 ์ดํ์ ๋น๋๋ฅผ ๊ฐ์ง๋ ์นดํ ๊ณ ๋ฆฌ๊ฐ ์๋ค๋ฉด, ๊ทธ๊ฒ์ ๋ค๋ฅธ ์นดํ ๊ณ ๋ฆฌ์ ํฉ์น๋ pooling์ ์ํํ๋ผ!
์์ ์์ ์์๋ ์นดํ ๊ณ ๋ฆฌ๊ฐ ๋จ 2๊ฐ์ธ ์ํฉ์ด์๋ค. ํ์ง๋ง, ์ฃผ์ฌ์ ๊ตด๋ฆฌ๊ธฐ์ ๊ฐ์ด ์นดํ ๊ณ ๋ฆฌ๊ฐ ์ฌ๋ฌ ๊ฐ์ธ ๊ฒฝ์ฐ๋ $\chi^2$ ๋ถํฌ์ DOF๊ฐ ๋ฌ๋ผ์ง๋ค. ๊ทธ ๊ณต์์ ์๋์ ๊ฐ๋ค.
Definition. Degree of Freedom for Goodness-of-fit
The degree of freedom $\nu$ = (#. of categories after pooling - 1) - #. of parameters estimated
(#. of categories)์์ $-1$์ ํ๋ ์ด์ ๋ Total value $n$์ด ์ฃผ์ด์ก๊ธฐ ๋๋ฌธ์ด๋ค. ๋ง์ง๋ง ์นดํ ๊ณ ๋ฆฌ์ ๊ฐ์ Deterministicํ๊ฒ ๊ฒฐ์ ๋๋ค!
ํต๊ณํ์์์ DOF์ ๋ํด ๊ถ๊ธํ๋ค๋ฉด, ์๋์ ํฌ์คํธ๋ฅผ ์ฝ์ด๋ณด๊ณ ์ค์!
๐ Degree of Freedom in Statistics
Test for Independence
<Chi-squared goodness-of-fit Test>๋ฅผ ์์ฉํด ๋ ๊ฐ์ ์นดํ ๊ณ ๋ฆฌ๊ฐ ์๋ก ๋ ๋ฆฝ(independent)์ธ์ง ๊ฒ์ ํด๋ณด์.
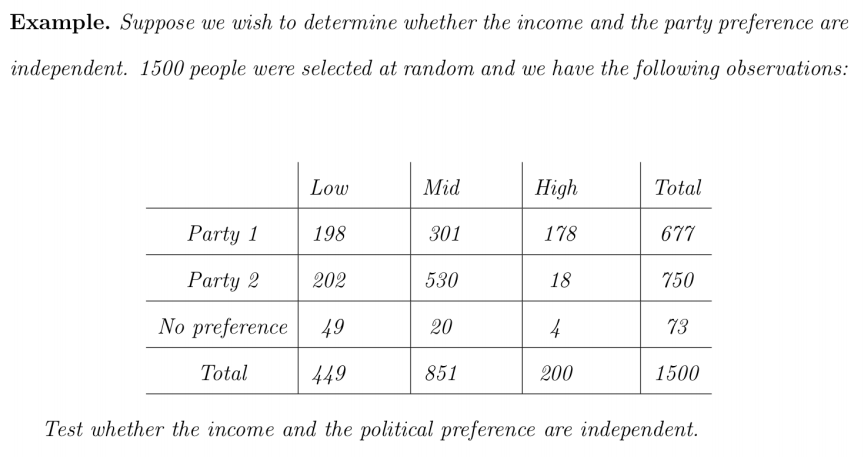
โincomeโ๊ณผ โpoliticalโ์ด ์๋ก ๋ ๋ฆฝ์ธ์ง๋ฅผ ๊ฒ์ ํด๋ณด์. ์๋์ ๊ฐ์ด $H_0$์ $H_1$์ ์ค์ ํ๋ค.
- $H_0$: income-political is independent
- $H_1$: they are not independent
$H_0$๋ฅผ ์์์ผ๋ก ํํํ๋ฉด ์๋์ ๊ฐ๋ค.
\[P(\text{party } 1 \; \And \; \text{low}) = P(\text{part } 1) \cdot P(\text{low})\]๋ ์นดํ ๊ณ ๋ฆฌ๊ฐ ๋ ๋ฆฝ์ด๋ผ๋ ๊ฐ์ $H_0$์์ ์ ๋ํ ์์ ๊ณต์์ ํ์ฉํ๋ฉด, ๊ฐ ์ํฉ์ expected value $e_{ij}$๋ฅผ ์ป์ ์ ์๋ค.
์๋ฅผ ๋ค์ด $e_{11}$์
\[\begin{aligned} e_{11} &= 1500 \times P(\text{P1} \; \And \; \text{Low}) \\ &= 1500 \times \frac{677}{1500} \times \frac{499}{1500} \\ &= \frac{677 \cdot 499}{1500} = 225.21 \end{aligned}\]์ด๋ฐ ๋ฐฉ์์ผ๋ก ๊ฐ entry์ ๋ํ expected value $e_{ij}$๋ฅผ ๊ตฌํ๋ค.
๋ค์์ผ๋ก chi-square test์ ๊ณต์์ $o_{ij}$, $e_{ij}$๋ฅผ ๋์ ํด $\chi^2$-value๋ฅผ ๊ตฌํ๋ค.
\[\chi^2 = \sum_{i=1}^3 \sum_{j=1}^3 \frac{(o_{ij} - e_{ij})^2}{e_{ij}}\]$\chi^2$ ๋ถํฌ์ DOF๋ ๊ตฌํด๋ณด๋ฉด,
\[\begin{aligned} \nu &= (9-1) - \left((3-1) + (3-1)\right) \\ &= 8 - (2 + 2) = 4 \end{aligned}\]์ด๋ โ(#. of parameters estimated) = $4$โ๊ฐ ๋๋ ์ด์ ๋ ๋ค์๊ณผ ๊ฐ๋ค.
์ฐ๋ฆฌ๊ฐ โpartyโ์ ๋ํ parameter๋ฅผ ๊ตฌํ๋ ค๋ฉด, ์ธ ๊ฐ์ง ๊ฒฝ์ฐ์ ๋ํ ํ๋ฅ ์ ๊ตฌํด์ผ ํ๋ค. ๊ทธ๋ฐ๋ฐ, ํ๋ฅ ์ ๊ฒฝ์ฐ ๅ์ด 1์ด ๋๊ธฐ ๋๋ฌธ์ ์ธ๊ฐ์ง ๊ฒฝ์ฐ ์ค ๋ ๊ฐ์ง ๊ฒฝ์ฐ๋ง ๊ตฌํ๋ฉด ๋๋ค. ๋ฐ๋ผ์, โpartyโ์ ๋ํด์ ๋ ๊ฐ์ง parameter๋ฅผ estimate ํด์ผ ํ๊ณ , ๋ง์ฐฌ๊ฐ์ง๋ก โincomeโ์ ๋ํด์๋ ๋ ๊ฐ์ง parameter๋ฅผ estimate ํด์ผ ํ๋ค. ๋ฐ๋ผ์, (#. of parameters estimated)๋ 4๊ฐ์ด๋ค.
์ด๊ฒ์ ๊ณต์์ผ๋ก ์์ฑํ๋ฉด ์๋์ ๊ฐ๋ค.
\[\begin{aligned} \nu &= r \cdot c - 1 - \left((r -1) + (c-1)\right) \\ &= r(c-1) - (c-1) \\ &= (r-1)(c-1) \end{aligned}\]$\chi^2$-value์ DOF $\nu$๋ฅผ ๊ตฌํ์ผ๋ฉด ๊ฒ์ ์ ์ํํ๋ฉด ๋๋ค.
Reject $H_0$, if $\chi^2 > \chi^2_{\alpha} (\nu)$.
Test for Homogeneity
์ด๋ฒ์๋ <Goodness-of-fit Test>๋ฅผ ์์ฉํด ๊ฐ ์นดํ ๊ณ ๋ฆฌ์์์ ๋ถํฌ๊ฐ ๊ท ์ผ(homogeneous)ํ์ง ๊ฒ์ ํด๋ณด์. ์๋ฅผ ๋ค๋ฉด, โ์ธ์ข ๋ณ๋ก ํก์ฐ์์ ๋นํก์ฐ์ ๋น์จ์ด ๋์ผํ๊ฐ?โ์ ๊ฐ์ ์ง๋ฌธ์ ๊ฒ์ฆํ๋ ๊ฒ์ด๋ค.
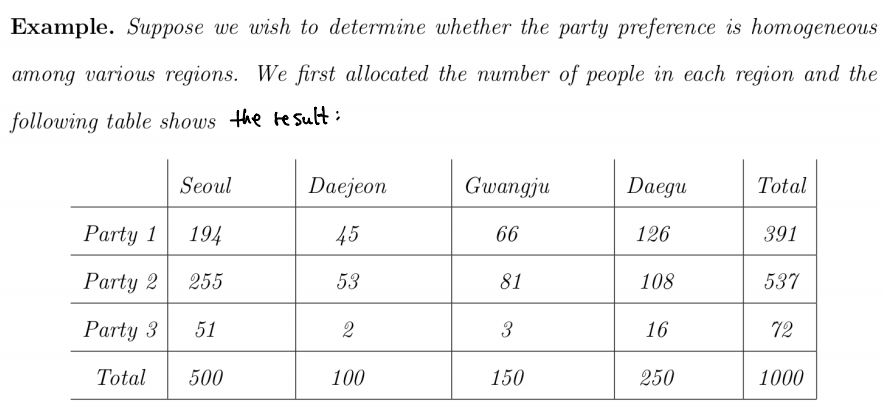
๋จผ์ ๋ฌด์์ ๊ฒ์ ํ๊ณ ์ ํ๋์ง ๋ช ํํ ์ ์ํด๋ณด์.
โIs the party preference homogeneous among various regions?โ
์ด๊ฒ์ ํ์ธํ๋ ค๋ฉด, โpart $i$โ์ ์ ํธํ๋ ๋น์จ์ด ๊ฐ ์ง์ญ๋ง๋ค ๋ชจ๋ ๋์ผํ์ง ํ์ธํด์ผ ํ๋ค. ์ด๊ฒ์ ์๋์ ๋ฑ์ ์ฑ๋ฆฝํจ์ ๋งํ๋ค.
\[P(\text{party } i \mid \text{Seoul}) = P(\text{part } i \mid \text{Daejeon}) = P(\text{party } i \mid \text{Gwangju}) = P(\text{party } i \mid \text{Daegu})\]์ด ๋ฑ์์ null hypothesis $H_0$๋ก ์ผ์ ๊ฒ์ ์ ์ํํ์!
์์ ํ๋ฅผ ๊ธฐ์ค์ผ๋ก $e_{11}$๋ฅผ ๊ตฌํด๋ณด์. ๋จผ์ โSeoulโ์ ์ด ์ธ๊ตฌ๋ 500์ด๋ค. ๊ทธ๋ฆฌ๊ณ ์ ์ฒด ์ฌ๋ ์ ์ค โparty 1โ์ ์ ํธํ๋ ์ฌ๋์ ๋น์จ์ 391/1000์ด๋ค. ๋ฐ๋ผ์, $e_{11}$์
\[e_{11} = 500 \times \frac{391}{1000}\]๋ง์ฐฌ๊ฐ์ง๋ก $e_{12}$์ ๊ฒฝ์ฐ๋ $e_{12} = 100 \times 391 / 1000$๋ก, $e_{21}$์ $e_{21} = 500 \times 537 / 1000$์ด๋ค.
์ฌ์ค Homogeneity Test๋ ์์์ ์ํํ Independence Test์ ๋์น์ด๋ค. Homogeneity Test์ $H_0$๊ฐ Inpendence๋ฅผ ์ง์ ์ ์ผ๋ก ํํํ์ง ์์์ง๋ง, ์ฝ๊ฐ ๋ณํํ๋ฉด Independence๋ก ์ ๋ํ ์ ์๋ค.
ํธ์๋ฅผ ์ํด $\text{party } i = B_i$, $\text{region } j = A_j$๋ก ํ์ํ๊ฒ ๋ค.
\[\begin{aligned} P(B_i \mid A_1) &= P(B_i \mid A_2) = x \\ \frac{P(B_i \cap A_1)}{P(A_1)} &= \frac{P(B_i \cap A_2)}{P(A_2)} = x \end{aligned}\]์ข๋ณ์ ๋ถ๋ชจ๋ฅผ ์ฐ๋ณ์ผ๋ก ๋๊ธฐ๋ฉด,
\[P(B_i \cap A_j) = x P(A_j)\]๊ฐ ๋๋๋ฐ, ์ด $P(B_i \cap A_j)$๋ฅผ ์ ๋ถ ๋ชจ์ผ๋ฉด โLaw of Total Probabilityโ์ ์ํด
\[P(B_i) = \sum_{j=1}^4 P(B_i \cap A_j) = x \cdot \cancelto{1}{\sum_{j=1}^4 P(A_j)} = x\]์ฆ, $x = P(B_i)$์ด๋ค. ์ด๊ฑธ ์ฒ์์ ์์์ ๋์ ํ๋ฉด,
\[P(B_i \mid A_1) = x = P(B_i)\]์ด๊ฒ์ $B_i$์ $A_j$๊ฐ ์๋ก ๋ ๋ฆฝ์์ ์๋ฏธํ๋ค!!! $\blacksquare$
์์ ์ฆ๋ช ์ ํตํด <Homogeneity Test>๊ฐ <Independence Test>์ ๋์น์์ ํ์ธํ๋ค. ๊ทธ๋์ <Independence Test>์์ ์ผ๋ ๊ฒ์ ๋ฐฉ์์ ๊ทธ๋๋ก ์ฐ๋ฉด ๋๋ค!!
DOF๋ <Independence Test>์ ๊ณต์์ผ๋ก ๊ตฌํด๋ณด๋ฉด,
\[\nu = (r-1) (c-1) = (3 - 1) (4 - 1) = 6\]๊ทธ๋ฆฌ๊ณ ๊ฒ์ ์ ์ํํ๋ฉด,
Reject $H_0$, if $\chi^2 > \chi^2_{\alpha}(\nu)$
Proportion Test and Chi-square Test
<chi-square test>๊ฐ โ<proportion test>์ ์ผ๋ฐํโ๋ผ๋ ๊ฑธ ์ค์ ๊ฐ๊ณผ ํจ๊ป ๋ค๋ค๋ณด๊ณ ์ ํ๋ค.
One Proportion Case
์๋ฉด์ ํ๋ฅ ์ด $p$์ธ p-coin์ด ์๋ค. ์๋์ ๊ฐ์ค์ ๊ฒ์ ํ๊ณ ์ ํ๋ค.
- $H_0$: $p = 1/3$
- $H_1$: $p \ne 1/3$
$20$๋ฒ์ ์คํ์ผ๋ก ์ป์ sample proportion์ $\hat{p} = 1/4$์๋ค.
One Proportion Test์ Statistic์ ์๋์ ๊ฐ๋ค.
\[\frac{p - \hat{p}}{\sqrt{p(1-p) / n}}\]์ด๊ฒ์ ๋์ ํด z-value๋ฅผ ๊ณ์ฐํ๋ฉด, $z = 0.791$์ด๋ค. Alternative Hypothesis $H_1$ ์ด ์์ธก ๊ฒ์ ์ ํํ์ด๋ฏ๋ก p-value๋ฅผ ๊ตฌํ๋ฉด, $0.428$์ด๋ค.
์ด๋ฒ์๋ chi-square GOF test๋ฅผ ํด๋ณด์. Test Statistic์ ์๋์ ๊ฐ๋ค.
\[\sum^2_{i=1} \frac{(o_i - e_i)^2}{e_i}\]์ด๊ฒ์ ๋์ ํด $\chi^2$-value๋ฅผ ๊ณ์ฐํ๋ฉด, $\chi^2 = 0.625$์ด๋ค. DOF $\nu = 1$์ด๋ฏ๋ก p-value๋ฅผ ๊ตฌํ๋ฉด, $0.429$์ด๋ค.
์์ฐ! ๋ ๊ฐ์ง ์ ๊ทผ ๋ชจ๋ ๋์ผํ p-value๋ฅผ ์ป์๋ค!!
Two Proportion Case
๋ ์งํฉ์ ๋น์จ์ด ๋์ผํ์ง, $p_1 = p_2$์ธ์ง๋ฅผ ๊ฒ์ ํ๊ณ ์ ํ๋ค. Test Statistic์ ์๋์ ๊ฐ๋ค.
\[\frac{\hat{p}_1 - \hat{p}_2}{\sqrt{\hat{p}\hat{q}(1/n_1 + 1/n_2)}}\]์ด๋, $\hat{p}$์ pooled proportion์ด๋ค.
\[\hat{p} = \frac{x_1 + x_2}{n_1 + n_2}\]z-value๋ฅผ ๊ณ์ฐํ๊ธฐ ์ํด ์คํ์ ๊ฐ์ ์์์๋ก ์ ํด๋ณด๋ฉด, $n_1 = 20$, $x_1 = 18$, $n_2 = 100$, $x_2 = 84$๋ผ๊ณ ํด๋ณด์.
์ด๊ฒ์ ๋์ ํด z-value๋ฅผ ๊ณ์ฐํ๋ฉด, $z = 0.686$์ด๋ค. ์์ธก ๊ฒ์ ์ ๋ํ p-value๋ฅผ ๊ตฌํ๋ฉด, $0.493$์ด๋ค.
์ด๋ฒ์๋ <Homogeneity Test>๋ก ์ ๊ทผํด๋ณด์. Test Statistic์ ์๋์ ๊ฐ๋ค.
\[\sum^2_{i=1} \sum^2_{j=1} \frac{(o_{ij} - e_{ij})^2}{e_{ij}}\]๋์ ํด์ $\chi^2$-value๋ฅผ ๊ณ์ฐํ๋ฉด, $\chi^2 = 0.471$์ด๋ค. ์ธํฐ๋ท์ ๋์๋ค๋๋ Independent Test Calculator๋ฅผ ์ฐ๋ฉด ๊ธ๋ฐฉ ๊ณ์ฐํ ์ ์๋ค! DOF $\nu = 1$์ด๋ฏ๋ก p-value๋ฅผ ๊ตฌํ๋ฉด, $0.493$์ด๋ค!
์์ฐ! ์ด๋ฒ์๋ ๋ ๊ฐ์ง ์ ๊ทผ ๋ชจ๋ ๋์ผํ p-value๋ฅผ ์ป์๋ค!
๋งบ์๋ง
๊ฒ์ (Testing)์ ๋ํ ๋ด์ฉ์ ์ฌ๊ธฐ๊น์ง๋ค!! ๐ ์ด๊ฒ์ผ๋ก โํต๊ณํ(Statistics)โ์ ๊ธฐ๋ณธ์ ์ธ ๋ด์ฉ์ ๋ชจ๋ ์ดํด๋ณธ ๊ฒ์ด๋ค!! ๐
๋ค์ ํฌ์คํธ๋ถํฐ <Simple Linear Regression>์ด๋ผ๋ ์๋ก์ด ์ฑํฐ๋ฅผ ์ดํด๋ณธ๋ค. ์ฃผ์ด์ง ๋ฐ์ดํฐ์์ โLinear Regressionโ์ ๊ณ์ $\beta_i$๋ค์ ์ด๋ป๊ฒ ์ฐพ์ ์ ์์์ง๋ฅผ ๋ค๋ฃจ๋ ์ฑํฐ๋ค!
๐ Introduction to Linear Regression