Predictive Distribution
โMachine Learningโ์ ๊ณต๋ถํ๋ฉด์ ๊ฐ์ธ์ ์ธ ์ฉ๋๋ก ์ ๋ฆฌํ ํฌ์คํธ์ ๋๋ค. ์ง์ ์ ์ธ์ ๋ ํ์์ ๋๋ค :)
๊ธฐํ ์๋ฆฌ์ฆ: Bayesian Regression
Bayesian Approach
์ด๋ฒ ํฌ์คํธ๋ถํฐ ๋ณธ๊ฒฉ์ ์ผ๋ก Bayesian Approach์ ๋ํด ํ๊ตฌํ๋ค. ๋จผ์ Bayesian์ ๊ด์ ์์๋ ํ๋ฅ (probability)์ โ๊ฐ์ค์ ๋ํ ๋ฏฟ์์ ์ ๋โ๋ก์ ์ดํดํ๋ค. ๊ทธ๋์ ์ฌ์ ๋ฏฟ์์ ๊ฐ์ง๊ณ ๊ฐ์ค์ ์ดํด๋ณด๊ณ , ์ดํ์ ๋ฐ์ดํฐ๋ฅผ ๊ด์ธกํ๋ค๋ฉด ๊ทธ ๋ฐ์ดํฐ๋ฅผ ๊ฐ์ง๊ณ ์๋กญ๊ฒ ๋ฏฟ์์ ๊ฐฑ์ ํด ์ฌํ ๋ฏฟ์์ ์ป๋๋ค. ์ด๋ฐ ์์ง-๊ฐฑ์ ์ ๊ณผ์ ์ ๋ฐ์ดํฐ๊ฐ ๋ฐ์ํ ๋๋ง๋ค ๊ณ์ ๋ฐ๋ณตํ๋ค.
๊ธฐ์ตํ ์ ์ Bayesian Approach๋ ํญ์ โ๋ถํ์ค์ฑ(uncertainty)โ์ ๋ํด ์๊ธฐํ๋ค๋ ๊ฒ์ด๋ค. ๊ณ ์ ์ ์ธ ํ๋ฅ ๋ก ์ด Point Estimation์ผ๋ก unbiased estimator ๋๋ the most efficient estimator of $\theta$1๋ฅผ ๊ตฌํ๊ฑฐ๋ ๋๋ Interval Estimation์ผ๋ก confidence level์ ๊ตฌํ๋ ๋ฑ์ ์ถ์ ์ ์ํํ์ง๋ง, Bayesian Approach๋ parameter $\theta$์ ๋ํ โํ๋ฅ ๋ถํฌโ๋ฅผ ์ฐพ๋ ๊ฒ์ ๋ชฉํ๋ก ํ๋ค. ๊ทธ๋์ Point Estimation์์ ์ฒ๋ผ parameter์ ๊ฐ์ $\theta = \theta_0$๋ก ํน์ ํ๋ ๊ฒ์ด ์๋๋ผ โ$\mu = 4$, $\sigma^2 = 1$์ธ ์ ๊ท๋ถํฌ๋ก parameter๊ฐ ๋ถํฌ๋์ด ์๋คโ๋ผ๊ณ ๋งํ๋ค.
Bayesian Approach์์๋ ๊ด์ธก ๋ฐ์ดํฐ๊ฐ ์ถ๊ฐ๋จ์ ๋ฐ๋ผ parameter์ distribution์ ๊ณ์ ๊ฐฑ์ ํ๋ค. ์ด๋ parameter์ prior distribution์ ์๋กญ๊ฒ ๊ด์ธก๋ ๋ฐ์ดํฐ๋ก ๊ฐฑ์ ํด posterior distribution์ ์ป๋ ์ ์ด๋ค. ์ด ์ํฐํด์์๋ ์ด๊ฒ์ โ๋ฐ์ดํฐ๊ฐ ํ๋ฅ ๋ถํฌ๋ฅผ ์ก์๋น๊ธฐ๋ ์์๊ณผ ๊ฐ๋คโ๊ณ ํํํ๋๋ฐ, ํํ์ด ๊ทธ๋ด์ธ ํ๋ค ๐ฒ ์์ธํ ๋ด์ฉ์ ํด๋น ์ํฐํด์ ์ ๋ถ๋ถ์ ์ ๊น ์ฝ์ด๋ณด๊ณ ์ค๋ ๊ฑธ ์ถ์ฒํ๋ค. ๊ธ์ ํตํด ๋ฐ์ดํฐ๊ฐ posterior distribution์ ์ด๋ป๊ฒ ๊ฐฑ์ ํ๋์ง ๊ทธ๋ฆฌ๊ณ prior distribution์ ์ ์ก๋๊ฒ ์ค์ํ ์ด์ ๋ฅผ ๊นจ๋ฌ์ ์ ์๋ค ๐
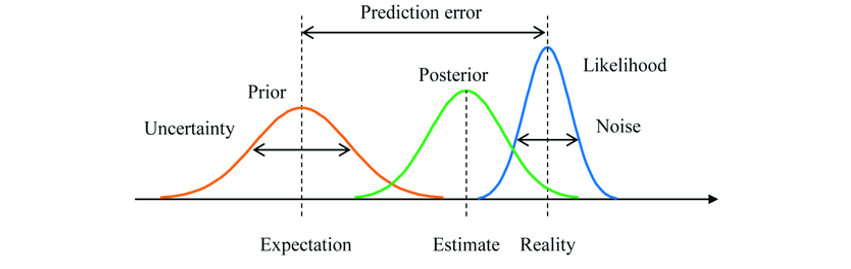
๊ธฐ์กด์ ๊ณ ์ ์ ์ธ ๋ฐฉ๋ฒ์ Point Estimator๋ confidence interval๋ฅผ ์ ๋ํ๋ค. ๊ทธ๋ฌ๋ Bayesian Approach์์๋ ๊ทธ๋ฐ ๊ฒ๋ค์ด ์ ํ ์์ผ๋ฉฐ๐ ๋จ์ง parameter์ ๋ํ posterior distribution์ ์ด์ฉํด ์๋ก์ด ๋ฐ์ดํฐ $x^{*}$๋ฅผ ์์ธกํ ๋ฟ์ด๋ค. ๊ทธ๋ฆฌ๊ณ ์ด ๊ณผ์ ์์ ๋ฑ์ฅํ๋ ๊ฒ์ด ๋ฐ๋ก <Predictive Distribution; ์์ธก ๋ถํฌ>์ด๋ค!
Parameter Posterior
์์ ๋ฌธ๋จ์์ Bayesian Approach๊ฐ ๊ด์ธก ๋ฐ์ดํฐ๋ก parameter์ distribution์ ๊ฐฑ์ ํ๋ค๊ณ ๊ธฐ์ ํ๋ค. ์ด๊ฒ์ ์ข๋ ์ดํด๋ณด์! ์ ์ผ ๋จผ์ parameter $\theta$์ ๋ํ prior distribution์ ๊ฐ์ ํ๋ค. ์ด๊ฒ์ <prior distribution of parameter> ๋๋ <parameter prior>๋ผ๊ณ ํ๋ฉฐ, ์ฌ๊ธฐ์๋ ์๋์ ๊ฐ์ ์ ๊ท ๋ถํฌ๋ฅผ ๊ฐ์ ํ๊ฒ ๋ค.
\[\theta \sim N(0, \tau^2 I)\]์ด์ ๊ด์ธก๋ ๋ฐ์ดํฐ $X = \{ x^{(1)}, \dots, x^{(m)} \}$๋ฅผ ์ด์ฉํด <parameter prior> $p(\theta)$๋ฅผ ๊ฐฑ์ ํด๋ณด์. <parameter posterior> $p(\theta \mid X)$๋ Bayes Rule์ ๋ฐ๋ผ ์๋์ ๊ฐ์ด ์ ๋ํ ์ ์๋ค.
\[\begin{aligned} p(\theta \mid X) &= \frac{p(X \mid \theta) p(\theta)}{p(X)} = \frac{p(X \mid \theta) p(\theta)}{\int_{\theta'} p(X \mid \theta') p(\theta') d\theta'} \\ &= \frac{p(\theta) \prod^m_{i=1} p(x^{(i)} \mid \theta)}{\int_{\theta'} p(\theta') \prod^m_{i=1} p(x^{(i)} \mid \theta') d\theta'} \end{aligned}\]์ด๋, likelihood์ $p(x^{(i)} \mid \theta)$๋ $\theta$๋ก parametized๋ ํ๋ฅ ๋ณ์ $X$์ ๋ํ ํ๋ฅ ๋ถํฌ๋ก ์ดํญ ๋ถํฌ, ์ ๊ท ๋ถํฌ, ํฌ์์ก ๋ถํฌ ๋ฑ๋ฑ์ด ๊ฐ๋ฅํ๋ค. likelihood๋ ๋ฐ์ดํฐ๊ฐ parameter $\theta$์ ์ํด ์ด๋ป๊ฒ parameterized ๋์ด ์์ ๊ฒ์ด๋ผ๊ณ ๊ฐ์ ํ๋ ๊ฒ์ด๊ธฐ ๋๋ฌธ์ ๊ฐฑ์ ํ๋ ๋์์ด ์๋๋ค! ๐
์ดํญ๋ถํฌ
\[p(x^{(i)} \mid \theta) = \frac{n!}{x!(n-x)!} \theta^x (1 - \theta)^{(n-x)}\]1D-์ ๊ท๋ถํฌ
\[p(x^{(i)} \mid \theta) = \frac{1}{\sqrt{2\pi} \sigma} \exp \left( - \frac{(x^{(i)} - \theta)^2}{2\sigma^2}\right)\]2D-์ ๊ท๋ถํฌ ($x^{(i)} \in \mathbb{R}^2$, also $\theta \in \mathbb{R}^2$)
\[p(x^{(i)} \mid \theta) = \frac{1}{\sqrt{2\pi} \sigma} \exp \left( - \frac{(x^{(i)} - \theta)^2}{2\sigma^2}\right)\]๋ฑ๋ฑ๋ฑ!!
Example. Parameter Posterior
๋์ ์ ๋์ก์ ๋, ์๋ฉด์ด ๋์ฌ ํ๋ฅ ์ด ๊ท ์ผ ๋ถํฌ๋ฅผ ๋ฐ๋ฅธ๋ค. ๋์ ์ ๋์ก๋๋ ์๋ฉด์ด ๋์์ ๋, parameter poster๋ฅผ ๊ตฌํ๋ผ.
Solution
โ์๋ฉด์ด ๋์ฌ ํ๋ฅ ์ด ๊ท ์ผ ๋ถํฌ๋ฅผ ๋ฐ๋ฅธ๋ค.โ
โ $p(\theta) = I_{(0 \le \theta \le 1)}$ (parameter prior)
โ๋์ ์ ๋์ง๋ค.โ
โ $p(x \mid \theta) = \theta^x (1 - \theta)^{(1-x)}$ (likelihood)
โ๋์ ์ ๋์ก๋๋ ์๋ฉด์ด ๋์์ ๋โ
โ $x_1 = 1$
๊ฐฑ์ ๋ parameter posterior์์๋ ์๋ฉด์ด ๋ง์ด ๋์ฌ ๊ฑฐ๋ผ๋ ํ๋ฅ (=๋ฏฟ์)์ด ๋ฐ์๋์๋ค.
$\blacksquare$
Predictive Distribution
<Predictive Distribution; ์์ธก ๋ถํฌ>๋ unobserved data $x^{*} \in X^{*}$์ ๋ํ prediction์ ์ํํ๋ ๊ณผ์ ์์ ์ ๋ํ๋ ๋ถํฌ์ด๋ค. ๊ทธ๋์ ์ด๋ฆ์ โpredictiveโ๋ผ๋ ์ด๋ฆ์ด ๋ถ์๋ค๊ณ ํ ์ ์๋ค. ๋ํผ์
์
๋๋ค <Predictive Distribution>์ parameter prior๋ก ์ ๋ํ๋์ง, observed data $X$๊ฐ ๋ฐ์๋ parameter posterior๋ก ์ ๋ํ๋์ง์ ๋ฐ๋ผ ๋ ๊ฐ์ง๋ก ๋๋๋ค.
Definition. Prior Predictive Distribution
Let $X = \{ x^{(1)}, \dots, x^{(m)} \}$ be a set of observed data, $X^{*}$ be a set of unobsersed data, and $x^{*} \in X^{*}$.
Then, the <prior predictive distribution> is
\[p(x^{*}) = \int p(x^{*}, \theta) d\theta = \int p(x^{*} \mid \theta) p(\theta) d\theta\]์ฆ, likelihood $p(x \mid \theta)$๋ฅผ parameter prior $p(\theta)$์ ํ๋ฅ ๋ถํฌ๋ฅผ ๊ณ ๋ คํด ์ ๋ถํ ๊ฒ์ด <prior predictive distribution>์ด๋ค.
๊ทธ๋ฌ๋ <prior predictive distribution>์ observed data $X$๋ฅผ ์ ํ ์ฐ๊ณ ์์ง ์๋ค. observed data๋ฅผ ์ ๋๋ก ํ์ฉํ๋ ค๋ฉด parameter posterior $p(\theta \mid X)$๋ก ์ ๋ํ <posterior predictive distribution>์ ์ฌ์ฉํด์ผ ํ๋ค!
Definition. Posterior Predictive Distribution
๋ณดํต $x^{*}$์ $X$๋ฅผ ๋ ๋ฆฝ์ด๋ผ๊ณ ๊ฐ์ ํ๊ธฐ ๋๋ฌธ์ ๋๋ iid๋ฅผ ๊ฐ์ ํ๋ฏ๋ก,
\[p(x^{*} \mid X) = \int p(x^{*} \mid \theta, X) p(\theta \mid X) d\theta = \int p(x^{*} \mid \theta) p(\theta \mid X) d\theta\]<prior predictive distribution>๊ณผ ๋น๊ตํ์ ๋ ๋ฌ๋ผ์ง ์ ์ ์ ๋ถ ๋ด๋ถ์ ํจ์๊ฐ parameter prior $p(\theta)$์์ parameter posterior $p(\theta \mid X)$๋ก ๋ฐ๋์๋ค๋ ์ ์ด๋ค! <posterior predictive distribution>์ ๊ด์ธก๋ ๋ฐ์ดํฐ๋ก ๊ฐฑ์ ๋ <parameter posterior>๋ฅผ ์ฌ์ฉํ๊ธฐ ๋๋ฌธ์ ์ค์ ๋ชจ์(parameter)์ ๊ทผ์ ํ ๊ฒ์ผ๋ก ๊ธฐ๋๋๋ ๋ถํฌ๋ฅผ ๋ฐํ์ผ๋ก ์์ธก(prediction)ํ๋ค๊ณ ๊ธฐ๋ํ๊ฒ ๋๋ค.
์ด ์ํฐํด์ ํด๋น ๋ถ๋ถ์์ predictive distribution์ ์ ๋ํ๋ ๊ฐ๋จํ ์์ ๋ฅผ ํ์ด๋ณผ ์ ์๋ค. ๋ฌธ์ ๊ฐ ์ข์ผ๋ ํ๋ฒ์ฏค ํ์ด๋ณด๋๋ก ํ์ ๐ ์ฐธ๊ณ ๋ก ์ฒซ๋ฒ์งธ ์์ ์์ Gamma function $\Gamma$๋ฅผ ์จ์ ์ ๋ถํ๋ ๋ถ๋ถ์ Beta Distribution์ ๋ํ ์ ๋ถ์ด๋ค.
๋ค์ ํฌ์คํธ์์๋ <Predictive Distribution>์ ์ด์ฉํด Regression Problem์ ๋ค๋ฃฌ๋ค. ์ด๊ฒ์ <Bayesian Linear Regression>์ด๋ผ๊ณ ํ๋ฉฐ ์ด๋ฒ ํฌ์คํธ๋ฅผ ์ ์ดํดํ๋ค๋ฉด ๋ค์ ํฌ์คํธ๋ฅผ ์ฝ๊ฒ ์ดํดํ ์ ์์ ๊ฒ์ด๋ค ๐
๐ Bayesian Regression
reference
- [๋ฒ์ญ] ์ ํ ํ๊ท ๋ชจ๋ธ Bayesians vs Frequentists
- Prior & Posterior Predictive Distributions
- ์ฌ์ ์์ธก๋ถํฌ์ ์ฌํ์์ธก๋ถํฌ(Prior and posterior predictive distribution)
- [Bayesian DL] 1. Properties of Gaussian Distribution and Prior(Posterior) Predictive Distribution
-
unbiased estimaor with the smallest varianceย ↩