F-distribution
โํ๋ฅ ๊ณผ ํต๊ณ(MATH230)โ ์์ ์์ ๋ฐฐ์ด ๊ฒ๊ณผ ๊ณต๋ถํ ๊ฒ์ ์ ๋ฆฌํ ํฌ์คํธ์ ๋๋ค. ์ ์ฒด ํฌ์คํธ๋ Probability and Statistics์์ ํ์ธํ์ค ์ ์์ต๋๋ค ๐ฒ
์๋ฆฌ์ฆ: Sampling Distributions
Definition. F-distribution
If $V_1 \sim \chi^2(n_1)$ and $V_2 \sim \chi^2(n_2)$ are independent,
then $F := \dfrac{V_1/n_1}{V_2/n_2}$ is called <Snedecorโs F-distribution>1 with degrees of freedom $n_1$ and $n_2$, and denoted as
\[F \sim F(n_1, n_2)\]ps) ์ผ๋ฐ์ ์ผ๋ก, $F(n_1, n_2) \ne F(n_2, n_1)$์ด๋ค. F-distribution์ non-symmetric์ด๋ผ๋ ๋ง.

Image from Wikipedia
Remark.
1. The order of $n_1$ and $n_2$ is very important.
In fact we have $F(n_1, n_2) \overset{D}{=} \dfrac{1}{F(n_2, n_1)}$.
2. Let $f_\alpha (n_1, n_2)$ be the number $x$ such that $\alpha = P\left(F(n_1, n_2) \ge x\right)$.
Here, we have $f_{1-\alpha}(n_1, n_2) = \dfrac{1}{f_{\alpha}(n_2, n_1)}$
Quick Proof.
๋ฐ๋ผ์,
\[\begin{aligned} \alpha &= P \left( F(n_2, n_1) > \frac{1}{f_{1-\alpha}(n_1, n_2)} \right) \\ &= P \left( F(n_2, n_1) > f_{\alpha}(n_2, n_1) \right) \end{aligned}\]๋ฐ๋ผ์,
\[f_\alpha (n_1, n_2) = \frac{1}{f_{1-\alpha}(n_2, n_1)}\]$\blacksquare$
Theorem.
Supp. we have two independent random samples $X_1, \dots, X_{n_1}$ from $N(\mu_1, \sigma_1^2)$ and $Y_1, \dots, Y_{n_2}$ from $N(\mu_2, \sigma_2^2)$.
Let $S_1^2 = \dfrac{\sum^{n_1}_{i=1} (X_i - \bar{X})^2}{n_1 - 1}$ and \(S_2^2 = \dfrac{\sum^{n_2}_{i=1} (Y_i - \bar{Y})^2}{n_2 - 1}\).
Note that $(n_1 - 1)S_1^2/\sigma_1^2 \sim \chi^2 (n_1 - 1)$ and $(n_2 - 1)S_2^2/\sigma_2^2 \sim \chi^2 (n_2 - 1)$.
Then,
\[F := \frac{S_1^2 / \sigma_1^2}{S_2^2 / \sigma_2^2} \sim F(n_1 - 1, n_2 - 1)\]Proof.
์ด๋, $\dfrac{(n_1 - 1) S_1^2}{\sigma_1^2} \sim \chi^2 (n_1 - 1)$์ด๋ฏ๋ก <F-distribution>์ ์ ์์ ๋ฐ๋ผ
\[F := \frac{S_1^2 / \sigma_1^2}{S_2^2 / \sigma_2^2} = \frac{V_1 / (n_1 - 1)}{V_2 / (n_2 - 1)} \sim F(n_1 - 1, n_2 - 1)\]Examples
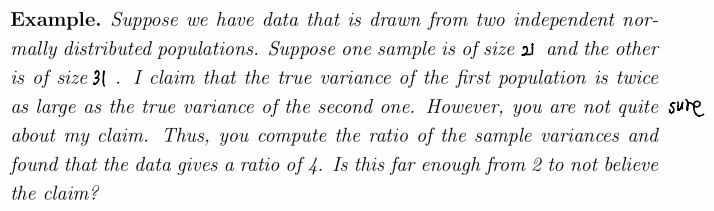
$n_1 = 21$, $n_2 = 31$
Claim: $\sigma_1^2/\sigma_2^2 = 2$ but, for sample variances, $S_1^2/S_2^2 = 4 > 2$.
\[\begin{aligned} &P\left(S_1^2/S_2^2 \ge 4 \quad \text{when} \quad \sigma_1^2/\sigma_2^2 = 2\right) \\ &= P \left( \frac{S_1^2 / \sigma_1^2}{S_2^2 / \sigma_2^2} \ge 4 \cdot \frac{1}{2} = 2 \right) \\ &= P(F(20, 30) \ge 2) \end{aligned}\]Here, $f_{0.05}(20, 30)=1.93$ and $f_{0.01}(20, 30) = 2.55$.
The the value of $2$ is btw $1.93$ and $2.55$.
Therefore,
\[P(F(20, 30) \ge 2) \in [0.01, 0.05]\]์ด๊ฒ์ ์๋ฏธ๋ sample variance์ ๋น์จ์ด 4๊ฐ ๋๋ ํ๋ฅ ์ ์ง๊ทนํ ๋ฎ๋ค๋ ๊ฒ์ด๋ค. ๊ทธ๋ฐ๋ฐ ์ด๊ฒ์ด ์ค์ ๋ก ๊ด์ธก๋์์ผ๋ฏ๋ก, ์ฐ๋ฆฌ์ ๊ฐ์ ์ธ $H_0: \sigma_1^2 / \sigma_2^2 = 2$๋ฅผ ๊ธฐ๊ฐํ๊ณ , ๋์ population variance์ ๋น์จ์ด ๋ ์ปค์ ธ์ผ ํ๋ค๋ ๋๋ฆฝ ๊ฐ์ค $H_1: \sigma_1^2 / \sigma_2^2 > 2$๋ฅผ ์ฑํํด์ผ ํ๋ค. $\blacksquare$
์ง๊ธ๊น์ง ์ฐ๋ฆฌ๋ population distribution์ parameter์ธ โํ๊ท โ๊ณผ โ๋ถ์ฐโ์ ๋ํด ์ถ์ ํ๋ค. ์ด์ด์ง๋ ํฌ์คํธ์์๋ sample๋ก๋ถํฐ ์ป๋ ๋ถํฌ์ธ <EDF; Empirical Distribution Function>์ผ๋ก๋ถํฐ population distribution์ ์ถ์ ํด๋ณธ๋ค. ์ด ๊ณผ์ ์์ ์ฐ๋ ๊ฒ์ด ๋ฐ๋ก <Quantile; ๋ถ์์>์ด๋ค!
๐ EDF and Quantile
-
โ[์ธ๋ค๋ฐ์ปค] F-๋ถํฌโ๋ผ๊ณ ์ฝ๋ ๊ฒ ๊ฐ๋ค.ย ↩