Proportion Estimation on Bernoulli Distribution
โํ๋ฅ ๊ณผ ํต๊ณ(MATH230)โ ์์ ์์ ๋ฐฐ์ด ๊ฒ๊ณผ ๊ณต๋ถํ ๊ฒ์ ์ ๋ฆฌํ ํฌ์คํธ์ ๋๋ค. ์ ์ฒด ํฌ์คํธ๋ Probability and Statistics์์ ํ์ธํ์ค ์ ์์ต๋๋ค ๐ฒ
Interval Estimation ํฌ์คํธ์์ ๋ค๋ฃฌ <Interval Estimation>์ ํน์ ์ํฉ์ ์ด๋ป๊ฒ ์ ์ฉ ํ๋์ง๋ฅผ ๋ค๋ฃจ๋ ํฌ์คํธ์ด๋ค. ์ง๊ธ๊น์ง์ ์ถ์ (Estimation)์ ๋ชจ๋ <Normal Distribution>์์ ์ถ์ถํ ์ํ์ ๋ํด ์ํํ๋ค. ์ด๋ฒ์๋ <Bernoulli Distribution>์ ์ํ์์ ์ถ์ ์ ์ํํ๋ค. ์ฆ, <Bernoulli Distribution>์ parameter์ธ ํ๋ฅ $p$๊ฐ ์ถ์ ์ ๋์์ธ ๊ฒ์ด๋ค!
Single Sample Estimation: Proportion Estimation
Supp. we have a p-coin. We want to verify that the coin is really a p-coin.
โจ Goal: Estimate $p$ based on the sample points!
Q1. Which coin estimator can we use for $p$?
A1. Here, we can use $\hat{p} = \dfrac{\text{# of heads}}{\text{# of toss}} = \dfrac{X_1 + \cdots + X_n}{n} = \bar{X}$.
Q2. How about 95% confidence interval for $p$?
A2. We can use CLT!!
\[\frac{\bar{X} - \mu}{\sigma / \sqrt{n}} = \frac{\hat{p} - p}{\sqrt{p(1-p)} / \sqrt{n}} \approx N(0, 1)\]then, the confidence interval is
\[\hat{p} - z_{\alpha/2} \cdot \sqrt{\frac{p(1-p)}{n}} \; \le \; p \; \le \; \hat{p} + z_{\alpha/2} \cdot \sqrt{\frac{p(1-p)}{n}}\]๐ฅ ํ์ง๋ง!!! ์์ ์์ ๋ฌธ์ ๊ฐ ์๋ค!! ๋ฐ๋ก $p$๋ฅผ ์ถ์ ํ๊ธฐ ์ํด interval์ ์ก์๋๋ฐ, interval์ ์ข์ฐ๋ณ์ ๋ $p$๊ฐ ๋ฑ์ฅํ๋ค๋ ๊ฒ์ด๋ค!! ๐ฒ
[Solution 1] solve the inequality for $p$.
โโ์ด์์คโ ๊ต์์ ์์ ๋ด์ฉ(ํ์์ ์ฉ)โ์ ์ฐธ๊ณ .
[Solution 2] replace $p$ by $\hat{p}$ // if $n$ is large, $\hat{p} \rightarrow p$ by LLN
Therefore, we the $n$ is large,
\[\hat{p} - z_{\alpha/2} \cdot \sqrt{\frac{\hat{p}(1-\hat{p})}{n}} \; \le \; p \; \le \; \hat{p} + z_{\alpha/2} \cdot \sqrt{\frac{\hat{p}(1-\hat{p})}{n}}\]์ด๋ฒ์๋ <Proportion Estimation>์ Error์ ๋ํด ์ดํด๋ณด์. Error๋ ์ด์ ์ Estimation๊ณผ ๋ง์ฐฌ๊ฐ์ง๋ก ์๋์ ๊ฐ์ด ์ ์๋๋ค.
The error $\left| \hat{p} - p \right|$ will not exceed $z_{\alpha/2} \cdot \sqrt{\dfrac{\hat{p}\hat{q}}{n}}$
Q. How large should the sample size be so that the error is at most $\epsilon$?
์ด๊ฒ์ Error์ ๋ํ ์์ $n$์ผ๋ก ๋ค์ ํ์ด์ ์ฝ๊ฒ ์ ๋ํ ์ ์์๋ค.
\[\begin{aligned} z_{\alpha/2} \cdot \sqrt{\frac{\hat{p}\hat{q}}{n}} &\le \epsilon \\ z_{\alpha/2}^2 \cdot \frac{\hat{p}\hat{q}}{n} &\le \epsilon^2 \\ \frac{(z_{\alpha/2})^2 \cdot \hat{p}\hat{q}}{\epsilon^2} &\le n \end{aligned}\]์ด๋, ์์ ์์ ๋ฌธ์ ๊ฐ ์๋ค!! ๋ฐ๋ก sample proportion $\hat{p}$๋ ์ฐ๋ฆฌ๊ฐ $n$์ ๊ฒฐ์ ํด ์ํ๋งํ๊ธฐ ์ ์๋ ๊ทธ ๊ฐ์ ์ ์ ์๋ค๋ ๊ฒ์ด๋ค!!!
[Solution 1] Guess $\hat{p}$, or use small size of sample to estimate $p$. From this, we get $\hat{p}$, and then use it!
[Solution 2] Consider the worst case, maximum error situation.
\[z_{\alpha/2} \cdot \sqrt{\frac{\hat{p}(1-\hat{p})}{n}} \le z_{\alpha/2} \cdot \sqrt{\frac{1}{4}\frac{1}{n}} \le \epsilon\] \[n \ge \left( \frac{z_{\alpha/2}}{2\epsilon} \right)^2\]Two Samples Estimation: Diff Btw Two Proportions
Two Samples Estimation: Diff Btw Two Means ํฌ์คํธ์์ ์ด๊ฒ๊ณผ ๋น์ทํ ์ํฉ์ ์ ํ ์ ์ด ์๋ค. ๊ทธ๋๋ Normal Distribution์์ ์ํํ๊ณ , sample variance $s^2$๋ฅผ ์ฐ๊ฒ ๋๋ฉด์, pooled sample variance $S_p^2$๋ <Welchโs t-test>๋ฅผ ์ํํ๋ค. ์์ ์ํฉ๊ณผ <Proportion Estimation>์ด ์ด๋ป๊ฒ ๋ค๋ฅธ์ง ๋น๊ตํ๋ฉด์ ์ดํด๋ณด์!
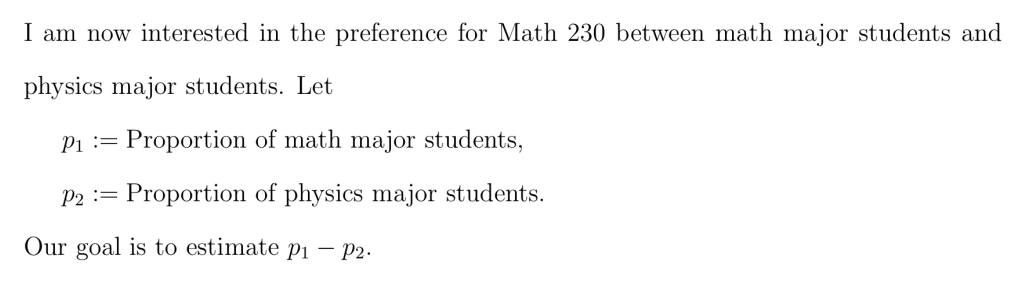
Select $n_1$: math major, and $n_2$: physics major, independently.
Assume $X_1, \dots, X_{n_1}$ and $Y_1, \dots, Y_{n_2}$ are two independent random samples.
From the sample, we get $\hat{p_1} = \bar{x}$, $\hat{p_2} = \bar{y}$. Then, $\hat{p_1} - \hat{p_2}$ can be estimator for $p_1 - p_2$.
By CLT,
\[\frac{(\hat{p_1} - \hat{p_2}) - (p_1 - p_2)}{\sqrt{p_1q_1/n_1 + p_2q_2/n_2}} \; \approx \; N(0, 1)\]์ด๋, ์์์ population proportion $p_1$, $p_2$ ๋ถ๋ถ์ sample proportion $\hat{p}_1$, $\hat{p}_2$๋ก ๋์ฒดํ๋ฉด,
\[\frac{(\hat{p_1} - \hat{p_2}) - (p_1 - p_2)}{\sqrt{\hat{p}_1\hat{q}_1/n_1 + \hat{p}_2\hat{q}_2/n_2}}\]Then, the $100(1-\alpha)\%$ confidence interval for $p_1 - p_2$ is
\[\left( (\hat{p_1} - \hat{p_2}) - z_{\alpha/2} \cdot \sqrt{\dfrac{\hat{p}_1\hat{q}_1}{n_1} + \dfrac{\hat{p}_2\hat{q}_2}{n_2}}, \; (\hat{p_1} - \hat{p_2}) + z_{\alpha/2} \cdot \sqrt{\dfrac{\hat{p}_1\hat{q}_1}{n_1} + \dfrac{\hat{p}_2\hat{q}_2}{n_2}} \right)\]Proportion Estimation and t-distribution
<Mean Estimation>์ ๊ธฐ์ต์ ๋ ์ฌ๋ฆฌ๋ฉด, population variance $\sigma^2$๋ฅผ ๋ชจ๋ฅด๊ธฐ์ sample variance $s^2$๋ฅผ ์ฐ๊ณ normal distribution $N(0, 1)$ ๋์ t-distribution $t(n)$๋ก ๊ทผ์ฌํ ๊ธฐ์ต์ด ์์ ๊ฒ์ด๋ค.
\[\frac{\bar{X} - \mu}{S / \sqrt{n}} \sim t(n-1)\]<Proportion Estimation>์์๋ populations proportion $p$์ ๊ฐ์ ๋ชจ๋ฅด๊ธฐ์ sample proportion์ธ $\hat{p}$๋ฅผ ๋์ ์ฌ์ฉ ํ๋ค.
\[\frac{\bar{X} - \mu}{\sigma / \sqrt{n}} = \frac{\hat{p} - p}{\sqrt{pq} / \sqrt{n}} = \frac{\hat{p} - p}{\sqrt{\hat{p}\hat{q}} / \sqrt{n}} \approx N(0, 1)\]๊ทธ๋ฌ๋ ์ด๋ฒ ๊ฒฝ์ฐ๋ t-distribution์ด ์๋๋ผ ๊ทธ๋๋ก normal distribution์ผ๋ก ๊ทผ์ฌํ์ฌ ์์ ์ป์๋ค. ์ ์ด๋ฒ ๊ฒฝ์ฐ์ t-distribution์ด ์๋ ๊ฑธ๊น?
<Mean Estimation>์์ ๊ฐ๋ณ sample $X_i$๊ฐ ์ ๊ท ๋ถํฌ๋ฅผ ๋ฐ๋ฅด๋ RV๋ผ๋ ๊ฐ์ ์ด ์๋ค.
\[X_i \sim N(\mu, \sigma^2)\]๊ทธ๋ฌ๋ <Proportion Estimation>์์ ๊ฐ๋ณ outcome $X_i$๊ฐ ๋์ ์๋ค ๊ฐ์ ์นดํ ๊ณ ๋ฆฌ ๋ณ์์ด๋ค. ๊ทธ๋ฆฌ๊ณ ์ด๊ฒ์ bernoulli distribution์ ๋ฐ๋ฅธ๋ค.
\[X_i \sim \text{Ber}(p)\]์ฆ, sample variable $X_i$์ด normal distribution sample์ด ์๋๊ธฐ ๋๋ฌธ์ sample proportion $\hat{p}$์ ์ฐ๋๋ผ๋ <t-distribution>์ผ๋ก ๊ทผ์ฌํ์ง ์๋ ๊ฒ์ด๋ค๋ผ๊ณ ์ดํดํ๊ณ ์๋ค. t-distribution์ ์๊ฐํ ์ ์ ๊ฐ ์ฑ๋ฆฝํ์ง๋ ๊ฒ์ด๋ผ๊ณ ๋ง์ด๋ค!
๋งบ์๋ง
์ง๊ธ๊น์ง
- population mean $\mu$
- population proportion $p$
์ ๋ํ ์ถ์ ์ ์ดํด๋ดค๋ค. ๋ค์ ํฌ์คํธ์์๋ sample variance $S^2$๋ก๋ถํฐ population variance $\sigma^2$๋ฅผ ์ถ์ ํ๋ ๋ฐฉ๋ฒ์ ๋ํด ์ดํด๋ณด๊ฒ ๋ค.
๐ Variance Estimation