Interval Estimation
โํ๋ฅ ๊ณผ ํต๊ณ(MATH230)โ ์์ ์์ ๋ฐฐ์ด ๊ฒ๊ณผ ๊ณต๋ถํ ๊ฒ์ ์ ๋ฆฌํ ํฌ์คํธ์ ๋๋ค. ์ ์ฒด ํฌ์คํธ๋ Probability and Statistics์์ ํ์ธํ์ค ์ ์์ต๋๋ค ๐ฒ
Introduction to Interval Estimation
Let $X_1, X_2, \dots, X_n$ be a random sample with $X_i \sim f(x; \theta)$, and $x_1, x_2, \dots, x_n$ be the values of the sample.
Here $\theta$ is unknown. The <interval estimation> is to find $(\hat{\theta}_L, \hat{\theta}_U)$ in which we expect to find the true value of the parameter $\theta$.
Q. How to find the interval?
A1. $\theta \in (-\infty, \infty)$ โ bad! ๐คฌ
A2. We would construct two estimators $\hat{\theta}_L$ and $\hat{\theta}_U$ from the random sample such that $P(\hat{\theta}_L < \theta < \hat{\theta}_U) = 1 - \alpha$.
Here, $(\hat{\theta}_L, \hat{\theta}_U)$ is called an <interval estimator> of $\theta$, $(\hat{\theta}_L, \hat{\theta}_U)$ is called a $100 \cdot (1 - \alpha)$% confidence interval. ๐ฅ
Also, $1-\alpha$ is called the <confidence coefficient> or <confidence level>. ๐ฅ
We usually take $\alpha = 0.01, \; 0.05, \; 0.1$.
๐ฅ Note that $(\hat{\theta}_L, \hat{\theta}_U)$ is not unique!! (๊ผญ ๋์นญ์ผ ํ์๋ ์๋ค๋ ๋ง)
Interval Estimation
์ด์ ์ํฉ์ ๋ฐ๋ฅธ <Interval Estimation> ๋ฐฉ๋ฒ์ ์ดํด๋ณด๊ฒ ๋ค!
- Estimate $\mu$ when $\sigma^2$ is known
- Estimate $\mu$ when $\sigma^2$ is unknown
z-value: Estimate $\mu$ when $\sigma^2$ is known
Example.
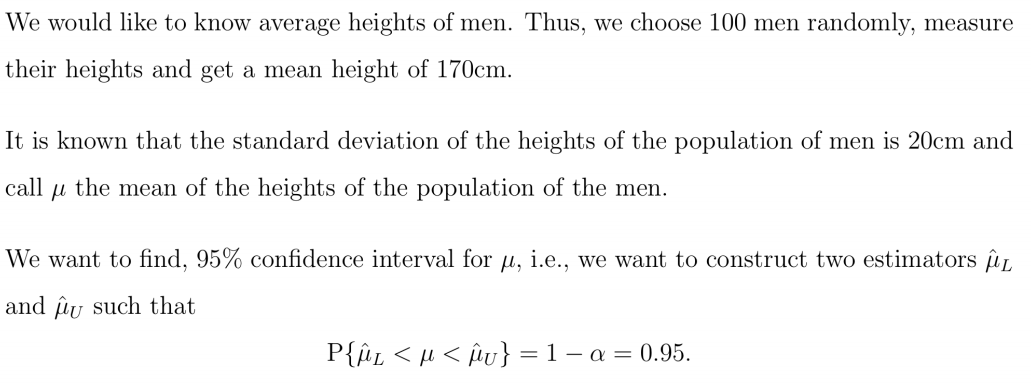
- $n=100$, $\bar{X} = 170$.
- $\sigma = 20$
1. We use $\bar{X}$ as a point estimator for $\mu$.
2. We can use CLT here.
\[\frac{\bar{X} - \mu}{\sigma / \sqrt{n}} \overset{D}{\approx} N(0, 1)\] \[\begin{aligned} 0.95 &= P(\hat{\mu}_L < \mu < \hat{\mu}_U) \\ &= P(-z_{0.025} \le z \le z_{0.025}) \\ &\approx P \left(-1.96 \le \frac{\bar{X} - \mu}{\sigma / \sqrt{n}} \le 1.96 \right) \\ &= P \left( \bar{X} - 1.96 \frac{\sigma}{\sqrt{n}} \le \mu \le \bar{X} + 1.96 \frac{\sigma}{\sqrt{n}} \right) \end{aligned}\]Here, we have $\hat{\mu}_L := \bar{X} - 1.96 \dfrac{\sigma}{\sqrt{n}}$, $\hat{\mu}_U := \bar{X} + 1.96 \dfrac{\sigma}{\sqrt{n}}$
$\therefore$ A 95% confidence interval would be $(170 - 3.92, 170 + 3.92)$.
Remark. Confidence Interval on $\mu$ when $\sigma^2$ is known
Let $x_1, \dots, x_n$ be given data points from a random sample $X_1, \dots, X_n$ with known population variance $\sigma^2$ and unknown population mean $\mu$.
If $\bar{x}$ is the sample mean, a $100(1-\alpha)\%$ confidence interval for $\mu$ is given by
\[\left( \bar{x} - z_{\alpha/2} \frac{\sigma}{\sqrt{n}} , \; \bar{x} + z_{\alpha/2} \frac{\sigma}{\sqrt{n}} \right)\]๐ฅ Note that this is an approximate interval unless $X_i \sim N(\mu, \sigma^2)$.
Q1. Is it true that $P \left( \mu \in (\hat{\mu}_L, \hat{\mu}_U) \right) \overset{?}{=} 0.95$? ๐ฅ
A1. No!! $\mu$ is not a random variable!
Q2. Then what does the confidence interval really mean?
A2. Its the number of counts that $\mu$ is actually belong to sampled interval! ๐ฅ
Let $x_1, \dots, x_n$ be a random sample. Supp. we obtain 1,000 samples and each sample has size $n$. Then, we have the following:
1st sample: $x_{11}, x_{12}, \dots, x_{1n}$ โ $\bar{x}_1$ โ confidence \((\bar{\mu}_{1L}, \bar{\mu}_{1U})\)
2nd sample: $x_{21}, x_{22}, \dots, x_{2n}$ โ $\bar{x}_1$ โ confidence \((\bar{\mu}_{2L}, \bar{\mu}_{2U})\)
$\vdots$
1000th sample: $x_{1000, 1}, x_{1000, 2}, \dots, x_{1000, n}$ โ $\bar{x}_1$ โ confidence \((\bar{\mu}_{1000, L}, \bar{\mu}_{1000, U})\)
์ด๋ ๊ฒ ์ป์ 1,000๊ฐ์ interval estimation์ ๋ํด, ์ ํํ 95%์ ๋น์จ, ์ฆ 950๊ฐ์ interval์ true parameter $\mu$๊ฐ ์ค์ ๋ก ํฌํจ๋์ด ์๋ค๋ ๋ง!
Error of Interval Estimation
Definition. Error of estimation
Now, letโs consider the error $| \bar{x} - \mu |$.
For an estimated interval $\left( \bar{x} - z_{\alpha/2} \frac{\sigma}{\sqrt{n}}, \bar{x} + z_{\alpha/2} \frac{\sigma}{\sqrt{n}}\right)$, the error is
\[\left| \bar{x} - \mu \right| \le z_{\alpha/2} \cdot \frac{\sigma}{\sqrt{n}}\]Theorem.
If $\bar{x}$ is used as an estimate of $\mu$, we can be $100(1-\alpha)\%$ confident that the error will not exceed $z_{\alpha/2} \cdot \frac{\sigma}{\sqrt{n}}$.
Theorem.
Q. How large can the sample size be if the error is at most $\epsilon$?
A. We want $\text{Err} = z_{\alpha/2} \cdot \frac{\sigma}{\sqrt{n}}$ to be less than $\epsilon$.
\[\text{Err} = z_{\alpha/2} \cdot \frac{\sigma}{\sqrt{n}} \le \epsilon\]Solve the inequality for $n$.
\[n \ge \left[ \frac{z_{\alpha/2} \cdot \sigma}{\epsilon} \right]^2\]One-sided Confidence Bounds
์ง๊ธ๊น์ง ์ฐ๋ฆฌ๋ ์ ๋์ ์ํฉ์ ์ดํด๋ณด๋ Two-sided Confidence Interval์ ์ดํด๋ณด์๋ค. ๊ทธ๋ฌ๋ ๋๋ก๋ ํ์ชฝ์ ์ํฉ๋ง ๊ด์ฌ์ ๋์์ด ๋ ์๋ ์๋ค! ๊ทธ๋์ ์๋์ ๊ฐ์ด One-side์ ๋ํ Confidence Interval์ ๊ตฌํด์ผ ํ ์๋ ์๋ค.
\[P(\hat{\theta}_L \le \mu) = 1 - \alpha\]์ฌ์ค two-sided์์ ์ฝ๊ฐ๋ง ์์ ํด์ฃผ๋ฉด ๋๋ค. two-sided์์์ Confidence Interval์ด ์๋์ ๊ฐ๋ค๋ฉด,
\[\bar{x} - z_{\alpha/2} \frac{\sigma}{\sqrt{n}} \; \le \; \mu \; \le \; \bar{x} + z_{\alpha/2} \frac{\sigma}{\sqrt{n}}\]์ฌ๊ธฐ์์ ํ์ชฝ๋ง ์ทจํด $\alpha$๋ฅผ ์ฌ์ฉํ๋ฉด ๋๋ค. ์ฆ,
\[\bar{x} - z_{\textcolor{red}{\alpha}} \frac{\sigma}{\sqrt{n}} \; \le \; \mu\]์ด๊ฒ์ ๊ณง
\[P \left(\bar{x} - z_{\textcolor{red}{\alpha}} \frac{\sigma}{\sqrt{n}} \; \le \; \mu \right) = 1 - \alpha\]์ ๊ฐ๋ค!
t-value: Estimate $\mu$ when $\sigma^2$ is unknown
์์์ ์งํํ๋ ๊ณผ์ ์ ๋ค์ ์ดํด๋ณด์. ์ฐ๋ฆฌ๋ CLT๋ฅผ ์ฌ์ฉํด sample mean $\bar{X}$๋ฅผ Normal ๋ถํฌ๋ก ๊ทผ์ฌํ๋ค.
\[Z = \frac{\bar{X} - \mu}{\sigma / \sqrt{n}}\]๊ทธ๋ฐ๋ฐ! population variance $\sigma$๋ฅผ ๋ชจ๋ฅด๋ ์ง๊ธ ์ํฉ์์๋ ์์ ๊ฐ์ด ์ ๊ทผํ ์ ์๋ค!! ๐ฒ $\sigma$๋ฅผ ๋ชจ๋ฅด๊ธฐ ๋๋ฌธ์ CLT ๊ทผ์ฌ์์ ๋ถ๋ชจ ๋ถ๋ถ์ $\sigma$๋ฅผ ์ธ ์ ์๊ธฐ ๋๋ฌธ์ด๋ค!
์ฐ๋ฆฌ๊ฐ ๊ทธ๋๋ง $\sigma^2$์ ๋น์ทํ๋ค๊ณ ์๊ฐํ๋ ๊ฒ์ด ์๋ค. ๋ฐ๋ก โsample varianceโ $S^2์ด๋ค! ์ด ๋ ์์ผ๋ก $\sigma$๋ฅผ ๋์ฒดํด ์์ ๋ค์ ์จ๋ณด์.
\[\frac{\bar{X} - \mu}{S / \sqrt{n}}\]์ด๋ผ! ์ด ์์ Studentโs t-distribution์์ ์ด๋ฏธ ์ดํด๋ณด์๋ค.
\[\frac{\bar{X} - \mu}{S / \sqrt{n}} \; \overset{D}{\sim} \; t(n-1)\]๊ทธ๋์ $t(n-1)$ distribution์์ $100(1-\alpha)\%$ confidence interval์ ๊ตฌํ๋ฉด,
\[P \left( -t_{\alpha/2} (n-1) < \frac{\bar{X} - \mu}{S / \sqrt{n}} < t_{\alpha/2}(n-1) \right) = 1 - \alpha\]๊ฐ ๋๋ค!
Remark. Confidence Interval on $\mu$ when $\sigma^2$ is unknown
Let $x_1, \dots, x_n$ be given data points from a normal random sample $X_1, \dots, X_n$ with mean $\mu$ and variance $\sigma^2$.
Here, the population mean $\mu$ and the population variance $\sigma$ are both unknown.
If $\bar{x}$ is the sample mean and $s^2$ is the sample variance, then a $100(1-\alpha)\%$ confidence interval for $\mu$ is given by
\[\left( \bar{x} - t_{\alpha/2}(n-1) \frac{s}{\sqrt{n}} , \; \bar{x} + t_{\alpha/2}(n-1) \frac{s}{\sqrt{n}} \right)\]Remark.
1. The width of the interval is random!
\[\left| \bar{x} - \mu \right| < t_{\alpha/2}(n-1) \cdot \frac{s}{\sqrt{n}}\]2. This confidence interval is not an approximation, since we assume sample $X_i$ as iid. normal $\mu$, $\sigma^2$.
Compare Point Estimator and Interval Estimator
Q. Does confidence interval give us more information about $\mu$ than a point estimator $\bar{x}$?
A. Not reallyโฆ ๐ค
[Point Estimator]
For a point estimator $\bar{x}$,
by LLN, $\bar{x} \rightarrow \mu$ as $n\rightarrow\infty$.
And the variance $\text{Var}(\bar{x}) = \sigma^2/n$.
[Interval Estimator]
For an interval estimator $\left(\bar{x} - z_{\alpha/2} \cdot \frac{\sigma}{\sqrt{n}}, \; \bar{x} + z_{\alpha/2} \cdot \frac{\sigma}{\sqrt{n}} \right)$,
the error is $\left\vert \bar{x} - \mu \right\vert \le z_{\alpha/2} \cdot \frac{\sigma}{\sqrt{n}}$.
๐ฅ This means the width of the confidence interval is determined by the standard deviation of the point estimator!! ์ด๊ฒ์ ๋ ์ค ํ Estimator๊ฐ ๊ฐ์ ๋๋ฉด, ๋ค๋ฅธ ํ๋์ ์ฑ๋ฅ๋ ๊ฐ์ ๋จ์ ๋งํ๋ค.
๋งบ์๋ง
์ด์ด์ง๋ ํฌ์คํธ๋ค์์๋ ์ํฉ๋ณ๋ก <Interval Estimation>์ ์ํํ๋ ๋ฐฉ๋ฒ์ ์ดํด๋ณผ ์์ ์ด๋ค! ๐คฉ
- Prediction & Tolerance Estimation
- Two Samples Estimation: Diff Btw Two Means
- Two Samples Estimation: Paired Observations
- Proportion Estimation
- Single Sample Estimation: Proportion Estimation
- Two Samples Estimation: Diff btw Two Proportions
- Variance Estimation
- Single Sample Estimation: Variance Estimation
- Two Samples Estimation: The Ratio of Two Variances