Reduction and NP-complete
2020-1ํ๊ธฐ, ๋ํ์์ โ์๊ณ ๋ฆฌ์ฆโ ์์ ์ ๋ฃ๊ณ ๊ณต๋ถํ ๋ฐ๋ฅผ ์ ๋ฆฌํ ๊ธ์ ๋๋ค. ์ง์ ์ ์ธ์ ๋ ํ์์ ๋๋ค :) ์ ์ฒด ํฌ์คํธ๋ Algorithm ํฌ์คํธ์์ ํ์ธํ์ค ์ ์์ต๋๋ค.
(Review) P and NP
์ ๊น ์ด์ ๋ด์ฉ์ ๋ณต์ตํด๋ณด์.
- $\textbf{NP}$: the class of all search problems
- $\textbf{P}$: the class of all search problems that can be solved in polynomial time.
๊ทธ๋ฆฌ๊ณ $\textbf{P} = \textbf{NP}$ ์ธ์ง $\textbf{P} \neq \textbf{NP}$ ์ธ์ง์ ๋ํ ๋ ผ์๋ ์ ๊น ๋ค๋ค๋ค. ๋๋ถ๋ถ์ ์ฐ๊ตฌ์๋ค์ $\textbf{P} \neq \textbf{NP}$๋ผ๊ณ ๋ฏฟ๊ณ ์๋ค. ๊ทธ๋ฌ๋ $\textbf{P} \neq \textbf{NP}$์์ ๋ฐํ๋ ๋ช ํํ ์ฆ๋ช ์ด ์๋ ๊ฑด ์๋๋ค. ๊ทธ๋ค์ ์ $\textbf{P} \neq \textbf{NP}$๋ผ๊ณ ๋ฏฟ๋ ๊ฑธ๊น?
๊ทธ๋ค์ โ์ฆ๊ฑฐโ๋ฅผ ๊ฐ์ง๊ณ ์๊ธฐ ๋๋ฌธ์ด๋ค! $\textbf{NP}$์ ์ํ๋ ๋ช๋ช search problem์ ๋ช์ญ๋ , ๋ช๋ฐฑ๋ ์ด ๊ฑธ๋ ค๋ ํจ์จ์ ์ธ ์๊ณ ๋ฆฌ์ฆ์ ์ฐพ์ ์ ์์๋ค๋ ๊ฒฝํ์ ์ธ ์ฆ๊ฑฐ๊ฐ ์๋ค. ๋, ํ๋์ ์ฆ๊ฑฐ๋ ํ์(Reduction)์ผ๋ก, ๊ทธ๋ฐ hardest problem๋ค์ด equivalent under reduction๋ผ๋ ๊ฒ์ด ์ฆ๋ช ๋์๊ธฐ ๋๋ฌธ์ด๋ค!
What reductions demonstrate is that the problems are all, in some sense, exactly the same problem, except that they are stated in different languages.
์ด๋ฒ ์ฑํฐ์์๋ $\textbf{NP}$์ hardest problem๋ค์ด ์ด๋ป๊ฒ equivalent under reduction ํ์ง ๊ฐ๋ณ๊ฒ ์ดํด๋ณด๊ฒ ๋ค.
Reduction

Definition. Reduction (search problem)
A reduction from search problem $A$ to search problem $B$ is a polynomial-time algorithm $f$ that transforms any instance $I$ of $A$ into an instance $f(I)$ of $B$.
Together with another polynomial-time algorithm $h$ that maps any solution $S$ of $f(I)$ back into a solution $S$ of $f(I)$ back into a solution $h(S)$ of $I$.
์ฆ, <Reduction>์ด๋ผ ํจ์
- instance $I$๋ฅผ ๋ณํ(transform) ํ๋ polynomial-time algorithm $f$
- $f(I)$์ solution $S$๋ฅผ $I$์ solution์ผ๋ก ๋ณํํ๋ polynomial-time algorithm $h$
์ฆ $f$์ $h$, 2๊ฐ์ง ์๊ณ ๋ฆฌ์ฆ์ด ํ์ํ ์์ ์ด๋ค!
๊ทธ๋ฐ๋ฐ <Reduction>์ ํ๋ ์ด์ ๊ฐ ๋ญ๊น? ๊ฑฐ๊ธฐ์ 2๊ฐ์ง ๋ชฉ์ ์ด ์๋ค.
- We know how to solve $B$ efficiently, and want to utilize it to solve $A$
- We know $A$ is hard, and use the reuction to prove $B$ is hard as well.
๋ณดํต์ ๊ฒฝ์ฐ๋ค์๋ ๋ชฉ์ 1์ ์ด๋ฃจ๊ธฐ ์ํด <Reduction> ์์ ์ ์ํํ๋ค. ๊ทธ๋ฌ๋ ์ด๋ฒ ๊ฒฝ์ฐ์๋ Problem B๊ฐ Problem A๋งํผ โ์ด๋ ต๋คโ๋ ์ฆ๋ช ํ๋ ๋ชฉ์ 2๋ฅผ ์ํด์ <Reduction> ์์ ์ ์ํํ๋ค!!
๋ณธ์ธ์ ์ฒ์์ ์์ ๋ฌธ์ฅ๋ค์ด ์ ์ดํด๊ฐ ์ ๋์๋ค. Reduction์ผ๋ก ์ ๋๋๋ Problem B์ Difficulty๊ฐ ๋ถ์์ฐ์ค๋ฌ์ ๋ณด์๊ธฐ ๋๋ฌธ์ด๋ค. ๋ณธ์ธ์ Reduction์ ๋ฌธ์ ๋ฅผ ํด๊ฒฐํ๊ธฐ ์ํ ์๋จ, ์ฆ ๋ชฉ์ 1์ ๋ํด์๋ง ์จ์ ํ ์ดํดํ๊ณ ์์๋ค.
๊ทธ๋ฌ๋ Wikipedia์ ๊ธฐ์ ๋ ์๋ ๊ตฌ์ ์ ์ฝ๊ณ ๋ชฉ์ 2๋ฅผ ์จ์ ํ ์ดํดํ ์ ์์๋ค.
Intuitively, problem A is reducible to problem B if an algorithm for solving B efficiently could also be used as a subroutine to solve problem A efficiently. When this is true, solving A cannot be harder than solving B. โHarderโ means having a higher estimate of the required computational resources in a given context.
์ฆ, problem A์์ problem B๊ฐ Reduction์ด ๊ฐ๋ฅํ ์๊ฐ๋ถํฐ Difficulty์ ๋ํ bound๊ฐ ์๊ธฐ๋ ์ ์ด๋ค. ๊ทธ๊ฒ์ โsolving A cannot be harder than solving Bโ๋ผ๋ ๋ฌธ์ฅ์ผ๋ก ํํ๋๋ค. ์ด๊ฒ์ Difficulty of B๊ฐ Difficulty of A์ upper bound๊ฐ ๋๋ค๋ ์ ์ด๋ฉฐ, ๋ฐ๊ฟ๋งํ๋ฉด Difficulty of A๊ฐ B์ lower bound๊ฐ ๋๋ค๋ ์ ์ด๋ค.
\[A \rightarrow B \implies \text{Dfficulty of A} \le \text{Difficulty of B}\]Reduction๋ฅผ ์์์ผ๋ก ํํ๋ฉด $A \rightarrow B$ ๋๋ $A \le_p B$๋ก ํํํ ์ ์๋ค. โ$B$ is polynomial-time reducible to $A$โ๋ผ๊ณ ์ฝ์ผ๋ฉด ๋๋ค. $A \le_m B$๋ ์๋๋ฐ, subscript๊ฐ $p$์ธ์ง $m$์ธ์ง์ ๋ฐ๋ผ mapping reduction, polynomial reduction์ผ๋ก ๋๋๋ค. ์ด์ ๋ํด์๋ ๋ณ๋์ ํฌ์คํธ์์ ๋ค๋ฃจ๋๋ก ํ๊ฒ ๋ค.
NP-complete
์ด์ <Reduction>์ด ๋ฌด์์ธ์ง ๋์ถฉ ์์์ผ๋ $\textbf{NP-complete}$์ ๊ฐ๋ ์ ์ ์ํด๋ณด๊ฒ ๋ค.
Definition. $\textbf{NP-complete}$
A search problem is $\textbf{NP-complete}$ if all other search problems reduce to it.
ํ ์คํธ์ ํจ๊ป ์๋ ๊ทธ๋ฆผ์ ์ดํด๋ณด์.
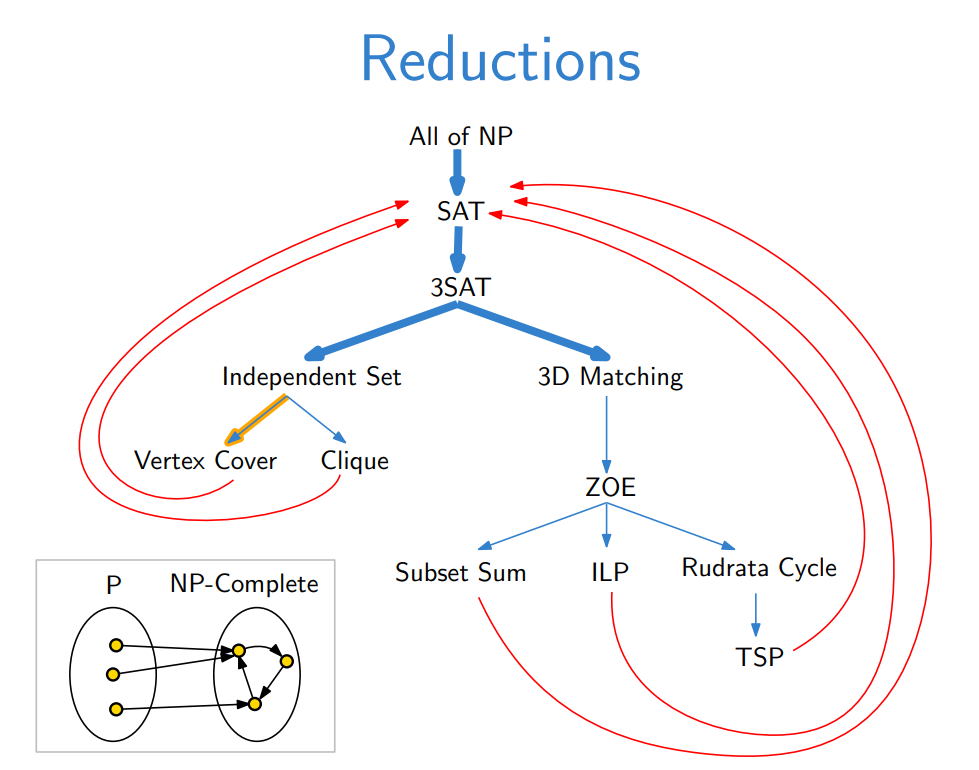
์ ๊ทธ๋ฆผ์ ๋ณด๋ฉด, ๋ชจ๋ Search Problem, ์ฆ $\textbf{NP}$ ๋ฌธ์ ๊ฐ <SAT>๋ก reduction ๋๋ ๊ฑธ ๋ณผ ์ ์๋ค. ๋ฐ๋ผ์ <SAT>๋ $\textbf{NP-complete}$๋ค. ๊ทธ์ธ์ ์ฐ๋ฆฌ๊ฐ ์ดํด๋ดค๋ <3-SAT>, <Independent Set Problem> ๋ฑ์ด ์ผ๋ จ์ Reduction์ ๊ฑฐ์ณ์ ๋ค์ <SAT>๋ก ํ์๋๋ค. ๋ฐ๋ผ์ ์ ๊ทธ๋ฆผ์ ๋ฑ์ฅํ๋ Search Problem ๋ชจ๋ $\textbf{NP-complete}$๋ค!
์ฌ์ค ์ ๊ทธ๋ฆผ์ ์ง์ ์ผ๋ก ์ดํดํ๋ ค๋ฉด, <Reduction>์ด composition property๋ฅผ ๊ฐ๋๋ค๋ ์ฌ์ค์ ์์์ผ ํ๋ค.
For reduction,
If $A \rightarrow B$ and $B \rightarrow C$, then $A \rightarrow C$
<Reduction>์ composition property๋ฅผ ํ ์ฉํ๋ฉด, โAll NP โ ???โ๋ฅผ ์ฆ๋ช ํ์ง ์๊ณ , โAll NP โ SATโ๋ฅผ ๋ณด์ธ ํ์ โSAT โ ???โ๋ฅผ ๋ณด์ด๋ฉด, โAll NP โ ???โ๋ฅผ ์ฆ๋ช ํ๋๋ฐ ์ถฉ๋ถํ๊ธฐ ๋๋ฌธ์ด๋ค!
๋ณธ์ธ ๊ต์ฌ์์๋ $\textbf{NP-complete}$๋ฅผ NP problem์ reduction ๊ฐ๋ฅ์ฑ์ผ๋ก ์ ์ ๋ด๋ ธ๋ค. ๊ทธ๋ฌ๋ ๋ค๋ฅธ ์๋ฃ์์๋ $\textbf{NP-hard}$๋ฅผ ์ ์ํ๊ณ , ์ด๋ฅผ ํ์ฉํด $\textbf{NP-complete}$๋ฅผ ์ ์ํ๋ค. ๊ทธ๋์ ์๋์ ๊ฐ์ Complexity Space๋ฅผ ์ ๋๋ก ์ดํดํ๋ ค๋ฉด, โNP-complete and NP-hardโ ํฌ์คํธ๋ฅผ ์ดํด๋ณด๋๋ก ํ์. ๋๋ Reduction์ ์ผ์ด์ค๋ค์ ๋จผ์ ์ดํด๋ณด๊ณ $\textbf{NP-complete}$๋ฅผ ์ดํดํด๋ ์ข๋ค.
์! ์ด์ ๋ช ํธ์ ํฌ์คํธ์ ๊ฑธ์ณ์ ์ด์ ๊ป ์ดํด๋ดค๋ $\textbf{NP}$ ๋ฌธ์ ๋ค์ด ์ด๋ป๊ฒ ์๋ก <Reduction> ๋๋์ง ์ดํด๋ณผ ๊ฒ์ด๋ค.